This is an automatically translated article.
Posted by Doctor Mai Vien Phuong - Department of Medical Examination & Internal Medicine - Vinmec Central Park International General Hospital
Image analysis using artificial intelligence, through advances such as machine learning or deep learning, is increasingly being applied to medical imaging. There has been considerable interest in using deep learning to detect various gastrointestinal disorders based on WCE imaging.
Part 1: Capsule endoscopy using artificial intelligence
1. Using Deep Learning to Classify Digestive Disorders
The most common indication for the use of WCE is evaluation of small bowel bleeding. WCE has also been used to diagnose other small bowel disorders, such as celiac disease, Crohn's disease, polyps, and tumors, to evaluate esophageal pathology in noncardiac chest pain, and to screen for bowel cancer. conclude.
Previous studies have focused on using deep learning to classify gastrointestinal diseases and lesions identified on WCE imaging. Not surprisingly, a frequently investigated outcome in the published literature is bleeding. Deep learning modeling has enhanced WCE's ability to detect bleeding lesions (including suspected blood content and vasodilatation) with relatively high sensitivity and specificity. In addition to bleeding, the researchers also used deep learning models in WCE to classify other gastrointestinal lesions such as ulcers, Crohn's disease, polyps, celiac disease, and hookworms.
Deep network architecture
Deep network architecture is a complete arrangement of neural networks in deep learning model including input layer, hidden layer and output layer. Although there are some variations with deep network architectures, 16 out of 17 studies used CNN-based architectures in their deep learning models. The choice of deep network architecture depends on the classification goal and each research group. However, many research groups prefer to use well-known CNN-based architectures when classifying WCE images or evaluating the performance of their custom deep learning architectures. These pre-built CNN-based architects include LeNet, AlexNet, GoogLeNet, VGG-Net, ResNet, RetinaNet, Single Shot MultiBox Detector, and Xception.
WCE capsule endoscope
In addition to variations in the deep learning architecture, the researchers had several variations in the WCE device. There are three brands of WCE devices mentioned in these deep learning studies: PillCam (Medtronics), NaviCam (Ankon Technologies), and MiroCam (IntroMedic). Deep learning models can be associated with each device. However, different devices have different raw image sizes and quality, brightness and camera angles. Because these devices are not standardized, applying a particular deep learning model may not perform with the same prediction accuracy when universally applied to other WCE devices.
Image resolution
Although the size and quality of the native WCE image is device dependent, image resolution depends on training time, deep network architecture, and lesion types. Intuitively, doctors prefer higher image resolution when making an image-based diagnosis. However, higher image resolution can lead to an increase in trainable parameters, floating point operations, memory requirements, and training time. To combat this, the original image is often modified (cropped or resized) to reduce the image resolution. The typical resolution range is 240 × 240 pixels to 320 × 320 pixels. It is worth noting that all studies using images taken by NaviCam (Ankon Technologies) chose a native image resolution of 480 × 480 pixels.
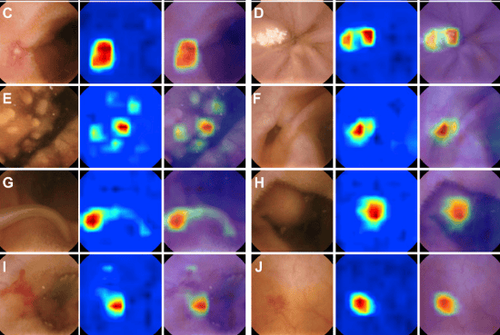
Data partitioning
The set of WCE images labeled by physicians as the primary data source, commonly referred to as a dataset. As part of the data pre-processing, the data set is usually divided into two groups. This generates two different datasets from the labeled WCE images. The first dataset is for the internal training and validation of deep learning models. After the final model is selected, a second dataset is used to test the model's performance against data that the model has not yet seen. Therefore, data partitioning is one of the factors that can impact the advance performance of deep learning models.
There are two common approaches to partitioning the initial data set identified during document review. The first is data partitioning based on composite images. The second is data partitioning per patient or video. The ratios of the two data sets vary depending on the study, but common ratios include 50:50, 70:30, and 80:20. The second approach to partitioning is often used when evaluating per-patient predictive performance. Therefore, we can see that the approach to data partitioning in WCE images is highly dependent on the study design.
Performance metrics
In the medical literature, the most common performance metrics are accuracy, sensitivity, specificity, and area under the curve (AUC). In the case of WCE imaging, where few WCE images are actual lesions, accuracy and specificity can be biased by deep learning models that accurately identify normal mucosa. For this reason, in data science, the focus of performance evaluation is on true positive classification. In other words, data scientists prefer their model to correctly classify small numbers of positive images (e.g., increased blood vessels, tumors, or ulcers) rather than correctly classifying mucosal images. normal fascia. Instead of precision and sensitivity, accuracy [true positive / (true positive + false positive)], recall [true positive / (true positive + false negative)] and F1 score (harmonized mean of accuracy and recall) is a common performance metric used by data scientists. It should be noted that accuracy and recall are also known as positive predictive value and sensitivity, respectively. Unfortunately, only a limited number of studies fully report these performance metrics sets, particularly F1 scores. In summary, it is important to consider performance metrics when defining or comparing the performance of deep learning models.
2. Using Deep Learning to Classify Objects Without Disease
The main goal when analyzing WCE images is to detect abnormalities in the gastrointestinal tract. However, detecting normal mucosa and anatomical landmarks is also useful. Currently, there are only two studies designed to classify subjects without the disease.
First study to use deep learning to classify complexity in the endothelial scene, including opacity, bubbles, clear blobs, wrinkles, and chemotaxis. Although these images may not contribute to the final diagnosis, they can be used to describe small bowel motility and help rule out negative imaging.
The second study created a predictive model to identify organ sites such as the stomach, intestines and colon. Organ classification can be used to calculate the transit time of WCE in each organ and to determine if there are any motility disturbances in the gastrointestinal tract.
An important aspect of physician evaluation for a WCE study is the identification of anatomical landmarks such as the first visualization of the stomach, duodenum, and cecum, which ultimately helps to calculate capsule transit time. through the small intestine. This transit time is important for determining the location of the lesion in the small intestine, which can help guide treatment with deep bowel endoscopic techniques.
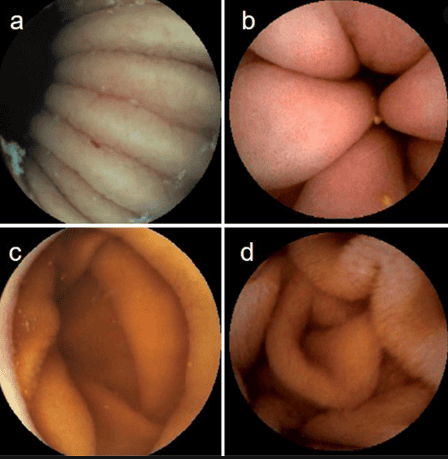
3. Uses of deep learning models in clinical practice
The ideal goal for WCE is to create a fully automated system for interpreting WCE images and generating reports that are accurate, at least comparable to conventional doctors' readings. Two retrospective studies compared the performance of regular reading with deep learning-assisted reading. The average reading time of the deep learning support reading in both studies was less than 6 minutes. Average typical reading time varies from 12 to 97 minutes depending on the expertise of the reader and the WCE reading range. In terms of overall lesion detection rate, there was a 3%-8% improvement in deep learning assisted reading compared to conventional reading.
Interestingly, the accuracy of the deep learning model (calculated during development) is higher than the actual detection rate. These findings may reflect real-world challenges affecting collaboration between humans and deep learning models. An additional limitation is that there is no clear definition of how read time is determined (e.g., from data preprocessing to final report generation).
Please dial HOTLINE for more information or register for an appointment HERE. Download MyVinmec app to make appointments faster and to manage your bookings easily.
References
Moglia A, Menciassi A, Schurr MO, Dario P. Wireless capsule endoscopy: from diagnostic devices to multipurpose robotic systems. Biomed Microdevices . 2007;9:235-243. [PubMed] [DOI] Li B, Meng MQ, Xu L. A comparative study of shape features for polyp detection in wireless capsule endoscopy images. Annu Int Conf IEEE Eng Med Biol Soc . 2009;2009:3731-3734. [PubMed] [DOI] Mishkin DS, Chuttani R, Croffie J, Disario J, Liu J, Shah R, Somogyi L, Tierney W, Song LM, Petersen BT; Technology Assessment Committee; American Society for Gastrointestinal Endoscopy. ASGE Technology Status Evaluation Report: wireless capsule endoscopy. Gastrointest Endosc. 2006;63:539-545. [PubMed] [DOI] Amporn Atsawarungruangkit, et al, Understanding deep learning in capsule endoscopy: Can artificial intelligence enhance clinical practice? Artif Intell Gastrointest Endosc. Oct 28, 2020; 1(2): 33-43
Please dial HOTLINE for more information or register for an appointment HERE. Download MyVinmec app to make appointments faster and to manage your bookings easily.