This is an automatically translated article.
Posted by Master, Doctor Mai Vien Phuong - Gastrointestinal Endoscopy - Department of Medical Examination & Internal Medicine - Vinmec Central Park International General Hospital.1. Identify real needs in daily practice
Artificial intelligence applications can take on tasks that are routine and often performed by pathologists, or provide new insights into diseases that human pathologists can't. Okay. These applications are needed to fill gaps and address unmet needs without disrupting day-to-day workflow in the pathology department. Needs include mitosis detection, calculation of tumor percentages, lymph node metastases, and other operations that are considered monotonous, repetitive, or vulnerable to inter-variation. higher server.2. The first step in the development of artificial intelligence applications
New medical artificial intelligence applications can be developed by many stakeholders, including pathologists, doctors, computer scientists, engineers, IT companies and companies medicine. However, views among experts in academia and industry differ. For example, individuals in academia and businesses have different goals, such as sponsorship grants, scholarly publications, and profitable commercial products.
Even if there is a problem that pathologists are eager to solve, the market size of the problem can be small. If the cost of developing an artificial intelligence application to solve a problem cannot be recouped by the subsequent profits from the sale of the application, the company may not develop it. There is a wide range of classification tasks in pathology, and it is difficult to secure a niche market for an AI application that specializes in a single task.
For example, an artificial intelligence algorithm can detect lymph node metastasis in breast cancer as reliably as human pathologists. However, the tool is not yet widely used or approved by regulatory agencies. Although, there can be many reasons and one of them is the imbalance between the overall cost of implementation and the benefits of detecting only breast cancer lymph node metastases in real life pathology practice. Another significant concern is obtaining consent for the use of patient data in artificial intelligence model development
Although, consent can be obtained for research use in most studies, but patients may not consent to the commercial use of their data necessary for product development, which can be an obstacle when developing products for clinical implementation.
Therefore, consent should be obtained at the start of research, conveying its commercial use for product development, a framework for global data sharing needs to be developed. To develop artificial intelligence algorithms, at least three parties need to cooperate, including pathologists who know the real need, academic experts who can develop the technology, and companies that will promote them. promote artificial intelligence applications as products. In addition, in order to achieve a sufficiently large market, it is important to develop global networks and online services using the cloud.
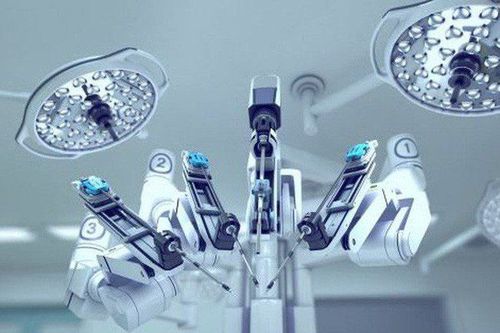
3. Development of artificial intelligence
After the concept of artificial intelligence has been conceived and cooperatively established, the development of artificial intelligence is carried out through the following steps: defining outputs, designing algorithms, collecting tracking samples pilot or larger, annotate and process the data, and perform statistical analysis of the data. Managing high-quality data sets is one of the major barriers in developing AI applications.
In general, CNNs require hundreds or thousands of datasets on pathology to achieve significant performance and lizability. For rare tumors, researchers can get a very limited number of images. Therefore, it requires effective data enhancement techniques and learning methods to solve this problem. In contrast, in the case of transfer learning, small scale datasets consisting of <100 digital slides may suffice. Also, publicly available datasets should be developed for global data sharing . However, very few such datasets are available in pathology, partly due to security, copyright, and financial issues. Even in such cases, TCGA provides many WSIs and related molecular data. However, even the TCGA data does not include a sufficient number of training cases for AI applications for clinical implementation. Another potential source of datasets could be the public challenges provided for the development of deep learning algorithms.
4. Major challenges for the implementation of artificial intelligence in practice
Developing artificial intelligence applications with sufficient performance requires training on huge datasets that demonstrate scanning capabilities and the ability to change staining protocols. The major challenges facing its implementation in practice are the laboratory infrastructure, reproducibility and robustness of the artificial intelligence model.
Recently, automatic methods to reduce blur in images have been developed. Automated algorithms (e.g. HistoQC and DeepFocus can report WSI quality standardization; these artificial intelligence applications automatically detect areas of optimal quality and remove out-of-focus areas or related to artifact.Standardization of color, shown by histopathology slides, is important for AI accuracy.Differences in color are often produced due to differences in appearance. lot or dye reagent manufacturer Variation in thickness of tissue sections, difference in staining procedure and difference in scanning characteristics
These variations lead to incomplete classification by artificial intelligence applications Artificial intelligence algorithms have been developed to normalize data, including staining capabilities and color characteristics.
5. Confirmation and regulation
As artificial intelligence-based technologies increasingly evolve, an evidence-based approach is required to validate them. Colling et al presented summary guidance under current in vitro device regulation and their recommendations for the key components of the validation process. In the medical laboratory, in addition to clinical evaluation, analytical validation should be considered. It is essential to establish steps and criteria for validating new tests against existing gold standards. To validate image analysis, this technique is often compared with "fundamental facts" (e.g., comparing artificial intelligence technology that analyzes HER2 expression in a tumor with a detailed assessment of a tumor that is analyzed by a tumor). done manually).
It would be appropriate to compare digital pathology techniques with the performance of human pathologists. However, given the inter-observer and intra-observer variability in the visual assessments of human pathologists, it is difficult to determine the underlying truth. It therefore involves careful design of the study and acceptance of the limitations of the current gold standard. Currently, most artificial intelligence applications seem to have difficulty establishing absolute ground truth.
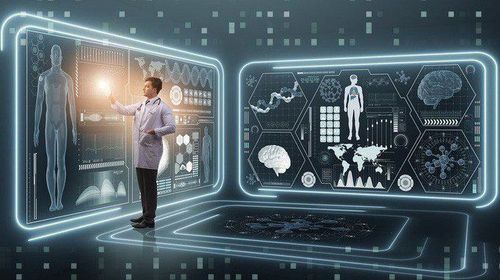
6. Implementation
Before implementing the application of artificial intelligence in real-life pathology practice, several obstacles must be resolved. Established business use cases and assurances from pathologists for the use of artificial intelligence systems need to be taken into account before significant investments of time, energy, and business are taken into account. costs for artificial intelligence applications and the necessary IT infrastructure. The changes needed to change the daily workflow in the pathology department, from slide to WSI, must be addressed. The department will require new pathology-related digital devices, a specific data management system, data storage facilities, and additional personnel to handle these changes. At the same time, institutional IT infrastructure is needed to enable users to operate through both on-premises and cloud-based systems. Thus, in the real world, digital pathology systems, which require significant investments, may hinder the implementation of these technologies. Notably, the enhancement microscope, which is connected directly to the cloud network service, can solve the problem of installing an entire slide scanner. Chen and colleagues report augmented reality microscopy, which overlays artificial intelligence-based information onto a sample view in real time, which could enable seamless integration of artificial intelligence into the process. work every day.
The enormous potential of artificial intelligence in pathology practice can be exploited by improving workflow, eliminating simple mistakes, increasing diagnostic reproducibility and revealing predictions not possible with the use of the usual visual methods of pathologists. Clinically deployed artificial intelligence applications are expected to be user-friendly, explainable, powerful, manageable, and cost-effective.
Please dial HOTLINE for more information or register for an appointment HERE. Download MyVinmec app to make appointments faster and to manage your bookings easily.
ReferencesYoshida H, Kiyuna T. Requirements for implementation of artificial intelligence in the practice of gastrointestinal pathology. World J Gastroenterol 2021; 27(21): 2818-2833 [DOI: 10.3748/wjg.v27.i21.2818]