This is an automatically translated article.
Post by Master, Doctor Mai Vien Phuong - Gastrointestinal endoscopy - Department of Medical Examination & Internal Medicine - Vinmec Central Park International General Hospital.Great advances of artificial intelligence (AI) in medical image analysis have been achieved in recent years. The integration of artificial intelligence is expected to cause a revolution in various fields of medicine, including gastrointestinal pathology such as stomach cancer and colorectal cancer.
1. Overview
Currently, deep learning algorithms have shown promising benefits in the fields of diagnostic histopathology, such as tumor identification, classification, prognostic prediction and biomarker prediction / genetic change. While AI cannot replace pathologists, carefully constructed medical AI applications can increase workforce productivity and diagnostic accuracy. in clinical practice.
Regardless of these promising advances, unlike the fields of radiology or cardiology, no histopathology-based artificial intelligence application has been approved by the regulator or for reimbursement public. Thus, it is implied that there are still some hurdles to overcome before artificial intelligence applications can be safely and effectively deployed in real-life pathology practice. Challenges were identified at various stages of the development process, such as needs identification, data management, model development, validation, regulation, daily workflow modifications, and more. date and cost-effective balance.
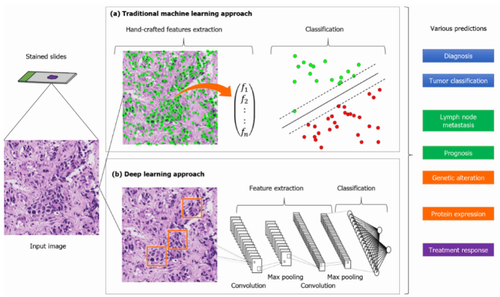
General process of building artificial intelligence models in pathology. Color slides are converted to digital input images using a slide scanner. Both (a) manual feature techniques and (b) deep learning methods produce categorical results, which are applied to clinically relevant predictions.
2. Application of artificial intelligence in histopathological diagnosis of gastric cancer
Several attempts have been made to classify the pathological images of gastric cancer using artificial intelligence. Before going into the details of the evaluation of artificial intelligence research, it should be noted that the performance comparison should not be based solely on accuracy, but we should pay attention to the difficulty of the task in the research framework, ie:
Dataset size (results for small sample sizes are less reliable) Resolution of detection (tissue or regional level), (3) number of classified categories Multiple site validation scores (train and test data sources are from the same site or not) Constraints for target lesions (eg, adenocarcinoma, or any lesion except lymphoma) .
3. Accuracy of artificial intelligence in histopathological diagnosis of gastric cancer
Sharma and colleagues documented gastric cancer detection on histopathology images using two methods based on deep learning: one that analyzed the morphological features of the entire image, while the other The other studies the focal features of the image independently. These models show an average accuracy of up to 89.7%. Iizuka et al. reported an artificial intelligence algorithm, based on CNN and recurrent neural network, to classify gastric biopsy images into gastric adenocarcinoma, adenocarcinoma, and adenocarcinoma. non-cancerous tissue. In three independent experimental datasets, the algorithm demonstrated an area under the curve (AUC) of 0.97 for the classification of gastric adenocarcinoma. Yoshida et al., using gastric biopsy samples, compared the classification results of experienced pathologists with the classification results of the machine learning-based "e-Pathologist" program developed by NEC Corporation. build. While the total concordance rate between them was only 55.6 percent (1702/3062), the concordance rate was as high as 90.6 percent (1033/1140) for biopsies that were negative for a single lesion. cancer. Tomita et al attempted to automate the identification of precancerous/neoplastic lesions in Barrett's esophagus or adenomas/gastric cancer.4. Application of artificial intelligence in histopathological diagnosis of colorectal cancer
As in gastric cancer, various artificial intelligence applications have recently been developed for colorectal cancer. Regarding tumor classification, several artificial intelligence algorithms have been trained to classify the dataset into two to six specific classes, such as normal, hyperplastic, adenoma, adenocarcinoma, and adenocarcinoma. histological subtypes of polyps or adenocarcinomas. Korbar et al. reported that the artificial intelligence model, built using more than 400 WSI, was able to classify five types of colorectal polyps with 93% accuracy. Wei et al demonstrated that the deep learning model, trained using WSI, can classify colorectal polyps, even in datasets from other hospitals, with reproducibility.
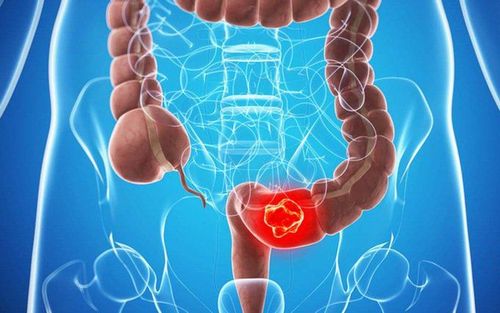
5. Performance of artificial intelligence models in histopathological diagnosis
Its accuracy is comparable to that of an on-site pathologist. While most studies show promising performance, an exact comparison of performance between these artificial intelligence applications is impossible and irrelevant. Each model is derived from different data sets with different annotations and focuses on different tasks. To accurately compare the performance of artificial intelligence models, it is necessary to have them perform a common task using a normalized data set with normalized annotations. Furthermore, several studies have predicted prognosis using pathologic imaging for colorectal cancer. Bychkov et al used 420 tissue microarray-WSIs to predict patient-specific 5-year survival and obtained an AUC of 0.69. Kather et al used more than 1000 histological images, collected from three institutions, to predict the patient's prognosis; they observed an accuracy of 99%.
Another study, using ResNet model to directly determine microscopic cell instability (MSI) on histological imaging, demonstrated an AUC of 0.77 for both FFPE and frozen samples from the Cancer Genome Map (TCGA). It is important to identify colorectal cancer with MSI; These tumors are reported to be highly responsive to immunomodulatory therapies. Furthermore, MSI may be a clue to the diagnosis of Lynch syndrome. MSI is usually identified by polymerase chain reaction (PCR), but not all patients are screened for MSI in clinical practice. Echle et al. recently developed a deep learning model for colorectal cancer detection with MSI using more than 8800 images. The deep learning algorithm demonstrated an AUC of 0.96 in the multi-organization validation cohort. In addition, the consensus molecular classification of colorectal cancer can be predicted from images of colorectal surgical specimens using CNN-based modeling.
Although prediction of molecular changes using artificial intelligence seems appealing, as clinically relevant biomarkers cannot be identified using HE-stained slides and conventional PCR testing is both costly and time consuming, especially artificial intelligence cannot achieve a full match with the gold standard test nor can it replace it. Therefore, users must consider how to use artificial intelligence to predict biomarkers with the right balance, cost-effectively in real life.
Please dial HOTLINE for more information or register for an appointment HERE. Download MyVinmec app to make appointments faster and to manage your bookings easily.
ReferencesYoshida H, Kiyuna T. Requirements for implementation of artificial intelligence in the practice of gastrointestinal pathology. World J Gastroenterol 2021; 27(21): 2818-2833 [DOI: 10.3748/wjg.v27.i21.2818]