This is an automatically translated article.
Posted by Master, Doctor Mai Vien Phuong - Gastrointestinal Endoscopy - Department of Examination & Internal Medicine - Vinmec Central Park International General HospitalDue to the rapid progression and poor prognosis of esophageal cancer (EC), the early detection and diagnosis of esophageal cancer is of great value in improving the prognosis for patients. Currently, science has applied artificial intelligence in risk stratification of early esophageal cancer.
Abbreviation:
EC: Esophageal cancer
AI: artificial intelligence
BE: Barrett esophagus
1. What is Artificial Intelligence?
Artificial Intelligence (AI: Artificial Intelligence) can be defined as a branch of computer science that deals with the automation of intelligent behaviors. AI is a part of computer science, and therefore it must be based on sound, applicable theoretical principles of the field.
2. What is the difference between artificial intelligence AI, Machine Learning and Deep Learning?
Artificial intelligence is the study of how to build machines capable of performing tasks that normally require human intelligence.
AI (Artificial Intelligence) covers many areas of research, from genetic algorithms to expert systems, and provides scope for arguments about what constitutes AI.
In the field of AI research, Machine Learning has achieved considerable success in recent years allowing computers to surpass or come close to matching human performance in areas ranging from facial recognition to speech and language recognition.
Machine Learning is the process of teaching a computer to do a task, instead of programming it how to do that task step by step. At the end of training, a Machine Learning system should be able to make accurate predictions when given the data. Machine Learning is often divided into supervised learning, where computers learn by example from labeled data, and unsupervised learning, where computers group similar data and pinpoint anomalies. .
Deep Learning is a subset of Machine Learning, capable of being different in some important respects from traditional shallow Machine Learning, allowing computers to solve a series of complex problems that cannot be solved .
In fact, a host of real-world problems do not fit such simple models. An example of one of these complex real-world problems is recognizing handwritten numbers.
To solve this problem, the computer needs to be able to deal with the great variety in the way data is presented. Each digit from 0 to 9 can be written in an infinite number of ways: the exact size and shape of each handwritten digit can vary greatly depending on the writer and under what circumstances.
Dealing with the variability of these features, and the greater interactive clutter between them, is where deep learning and deep neural networks come in handy.
Neural networks are structured mathematical models loosely inspired by the brain. Each neuron in a neural network is a mathematical function that takes data through the input, transforms that data into a more tunable form, and then spits it out through the output.
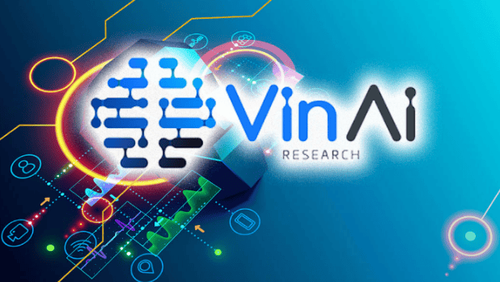
3. Overview of the application of artificial intelligence to gastrointestinal endoscopy
Due to the rapid progression and poor prognosis of esophageal cancer (EC), the early detection and diagnosis of esophageal cancer is of great value in improving the prognosis for patients. However, early endoscopic detection of esophageal cancer, especially Barrett dysplasia or esophageal squamous dysplasia, is difficult. Therefore, the demand for more effective methods of detecting early esophageal cancer features has led to intensive research in the field of artificial intelligence (AI). Deep learning and memory techniques (DL) have brought breakthroughs in image, video and other aspects of processing, while complex neural networks (CNN) have paved the way for detect high-resolution endoscopic images and videos. Many studies on complex neural networks in early esophageal cancer endoscopic image analysis show excellent performance including sensitivity and specificity and gradual progression from in vitro imaging analysis. for grading to real-time detection of early esophageal tumors in practice.
As AI technology enters pathology, contour lesions that are difficult to identify by endoscopists may become easier than before. In genomics, since genomic markers lack tissue specificity, they can now only be used as complementary measures. In predicting cancer risk, there is still a lack of prospective clinical studies to confirm the accuracy of risk stratification models.
4. Introduction to esophageal cancer
Esophageal cancer (EC) is the eighth most common cancer and the sixth leading cause of cancer death worldwide. Esophageal cancers mainly include esophageal adenocarcinoma (EAC) and esophageal squamous cell carcinoma (ESCC). Esophageal adenocarcinoma is the most common disease in Western countries, more than 40% of patients with esophageal adenocarcinoma are diagnosed after the disease has metastasized, and the 5-year survival rate is less than 5 years. 20%.
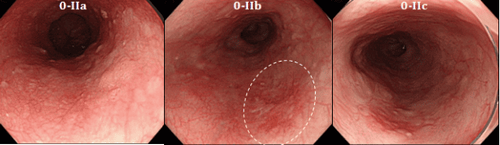
Although the incidence of esophageal adenocarcinoma is increasing globally, esophageal squamous cell carcinoma remains the most common (80%) of all esophageal cancers with the highest incidence on the "cancer belt" extending from East Africa and through the Middle East to Asia. Only 20% of patients with esophageal squamous cell carcinoma survive longer than 3 years, mainly due to late-stage diagnosis. In low-resource areas, the 5-year survival rate is much lower at around 3.4%. Early diagnosis can significantly improve outcomes for all esophageal cancers.
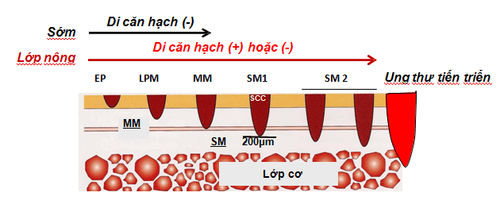
5. The role of esophagogastroduodenoscopy in the diagnosis of early esophageal cancer
Gastroscopy remains the primary way to detect early ESCC. However, the endoscopic features of these early lesions are subtle and easily missed compared with conventional white light endoscopy (WLE). Endocardial capillary loops (IPCLs), which are microvasculatures, are considered to be hallmarks of ESCC esophageal squamous cell carcinoma, because their morphological changes correlate with depth of invasion of the esophageal squamous cell carcinoma. esophageal squamous cell carcinoma.
Advanced endoscopic imaging modalities, such as endoscopic narrow band imaging (NBI), combined with magnified endoscopy, help improve imaging of cellular microvascular models in real mucosa management of patients with esophageal squamous cell carcinoma. Although NBI has shown high sensitivity in detecting esophageal squamous cell carcinoma, its effectiveness in identifying these lesions is still limited.
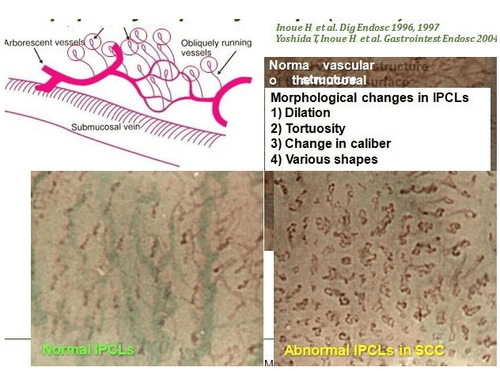
6. Application of Machine learning and Deep learning algorithms in esophageal cancer
The demand for more effective methods of early detection and characterization of esophageal cancer has led to intensive research in the field of artificial intelligence (AI). This can be determined by intelligence established by machines as opposed to the natural intelligence displayed by humans and other animals. Machine learning (ML) and deep learning (DL) are important parts of AI. Machine learning can be divided into supervised and unsupervised methods. Unsupervised learning is to identify groups in data according to commonalities, lacking knowledge of the number of groups or their meanings.
When the training package contains input-output pairs, a supervised learning model is required to map the new input to the output. Conventional ML techniques are limited in their ability to process natural data in its raw form. In the early stages of research and development, model training was mainly with ML, through which researchers had to manually extract probable disease features based on clinical knowledge. The power of this computer-aided diagnosis (CAD) system is weak and insufficient to be applied in clinical real-time diagnosis.
7. The Role of Convolutional Neural Networks (CNNs)
Convolutional Neural Network (CNN) is a supervised machine learning model inspired by the visual cortex of the human brain that processes and recognizes images. Each artificial neuron is a computational unit and all of them are interconnected, forming a network. Using multiple network layers, CNN can extract key features from an image with minimal pre-processing and then provide final classification through fully connected layers as output.
The increasing competition for performance has led to the increasing complexity of pooling layers leading to the concept of deep learning. The important aspect of deep learning is that these feature classes are not designed by human engineers. They are learned from data using a general-purpose learning process. Deep learning has brought breakthroughs in image, video and other aspects of processing, while iterative CNNs have shed light on the detection of endoscopic images and videos.
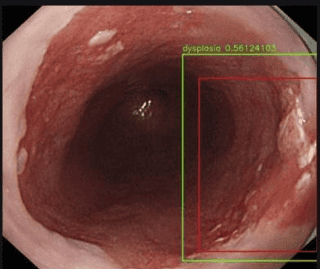
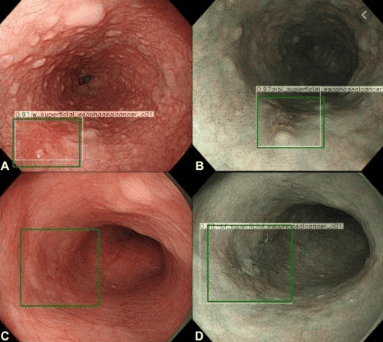
8. The role of artificial intelligence AI in risk stratification of early esophageal cancer
It is estimated that only 0.12% -0.43% of patients with Barrett's esophagus may progress to early esophageal cancer each year, it is particularly important to establish an effective model to predict the risk of esophageal cancer. of early esophageal cancer each year in patients with Barrett's esophagus. Previously risk stratification was based primarily on the presence of dysplasia, but its efficacy was not ideal.
Critchley-Thorne et al established a predictive model based on characteristic differences in immunofluorescence markers and histopathology between Barrett's esophagus patients who developed esophageal cancer. early each year and who do not have the disease.
Outcomes are not encouraging, with more than 30% of Barrett's esophagus patients developing early esophageal cancer each year classified as low-risk. Li et al established a prediction model based on single nucleotide polymorphism differences in the biopsied tissues of Barrett's esophagus patients with good performance to predict early esophageal cancer each year.
Unlike esophageal cancer per year, esophageal squamous cell carcinoma has no recognized precancerous disease, and endoscopic screening of the general population is clearly impractical. Therefore, it will be the focus of clinical screening to establish an effective model to predict which individuals are more likely to be diagnosed with esophageal squamous dysplasia. Etemadi et al.'s model based on epidemiological data from patients diagnosed with esophageal squamous dysplasia and healthy controls showed poor performance of this model. Moghtadaei et al.'s predictive model is based on better epidemiological data than Etemadi. Unfortunately, there is a lack of follow-up studies to verify the true accuracy of these models.
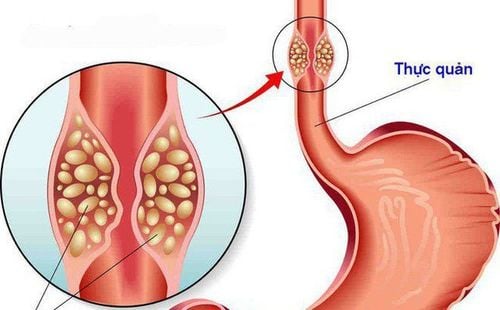
Artificial intelligence AI is trying to be used for endoscopic detection, pathological diagnosis, genetic diagnosis and cancer risk prediction of early esophageal cancer. It is useful for endoscopists and pathologists to improve diagnostic accuracy and assist physicians in treatment and follow-up strategies. Boundary lesions of esophageal cancer pathology are still difficult to define which could be the main direction of AI-assisted pathology diagnosis in the future. Genotyping can only be used as an adjunct to endoscopy and pathology until specific markers of EC gene diagnosis have been established. Greater accuracy of the CAD system in identifying lesions is required to improve diagnostic accuracy and enhance the accuracy of the risk stratification model which can be beneficial for risk prediction. risk of esophageal cancer.
Currently, gastrointestinal cancer screening is a scientific and effective measure for early detection of gastrointestinal cancer (esophageal cancer, stomach cancer, colon cancer) and giving a treatment plan. best treatment. Currently, Vinmec International General Hospital has a package of screening and early detection of cancers of the gastrointestinal tract (esophagus - stomach - colon) combined with clinical and paraclinical examination to bring the most accurate results. maybe.
When screening for gastrointestinal cancer at Vinmec, you will receive:
Gastrointestinal specialty examination with an oncologist (by appointment). Gastroscopy and colonoscopy with an NBI endoscope under anesthesia. Complete peripheral blood cytology (by laser counter). Automated prothrombin time test. Automated thrombin time test. Activated Partial Thromboplastin Time (APTT) test using an automated machine. General abdominal ultrasound For direct consultation, please dial HOTLINE or register online HERE.
Please dial HOTLINE for more information or register for an appointment HERE. Download MyVinmec app to make appointments faster and to manage your bookings easily.
References
1. Bray F , Ferlay J, Soerjomataram I, Siegel RL, Torre LA, Jemal A. Global cancer statistics 2018: GLOBOCAN estimates of incidence and mortality worldwide for 36 cancers in 185 countries. CA Cancer J Clin . 2018; 68 :394-424. [PubMed] [DOI]
2. Hur C, Miller M, Kong CY, Dowling EC, Nattinger KJ, Dunn M, Feuer EJ. Trends in esophageal adenocarcinoma incidence and mortality. Cancer . two thousand and thirteen; 119 :1149-1158. [PubMed] [DOI]
3. Thrift AP . The epidemic of oesophageal carcinoma: Where are we now? Cancer Epidemiol. 2016; 41 :88-95. [PubMed] [DOI]
4. Lu-Ming Huang, Wen-Juan Yang, Zhi-Yin Huang, Cheng-Wei Tang, Jing Li, Artificial intelligence technique in detection of early esophageal cancer, World J Gastroenterol. Oct 21, 2020; 26(39): 5959-5969