This is an automatically translated article.
Posted by Master, Doctor Mai Vien Phuong - Gastrointestinal Endoscopy - Department of Medical Examination & Internal Medicine - Vinmec Central Park International HospitalCurrently, artificial intelligence (AI) is becoming more and more sophisticated and brings higher efficiency and low cost. According to the assessment, AI promises to bring great potential in the field of health care, especially in genetic diagnosis of early esophageal cancer.
1.What is Artificial Intelligence? Artificial Intelligence (AI: Artificial Intelligence) can be defined as a branch of computer science that deals with the automation of intelligent behaviors. Artificial intelligence is a part of computer science and therefore it must be based on solid, applicable theories and principles of the field.
2. What is the difference between artificial intelligence AI, Machine Learning and Deep Learning? Artificial intelligence is the study of how to build machines capable of performing tasks that normally require human intelligence. Artificial Intelligence covers many areas of research, from genetic algorithms to expert systems, and provides scope for arguments about what constitutes artificial intelligence.
Machine Learning is the process of teaching a computer to perform a task, instead of programming it how to perform that task step by step. Machine Learning is often divided into supervised learning, where computers learn by example from labeled data, and unsupervised learning. In it, the computer groups similar data and pinpoints the anomaly.
Deep Learning is a subset of Machine Learning, capable of being different in some important respects from traditional shallow Machine Learning, allowing computers to solve a series of complex problems that cannot be solved .
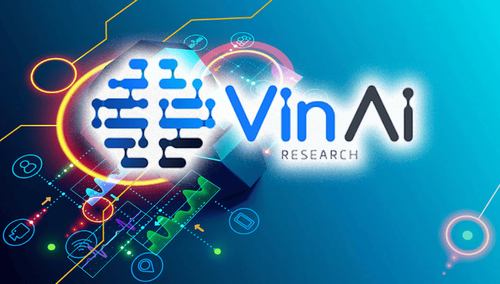
Hình 1: Trí tuệ nhân tạo AI ngày càng được ứng dụng nhiều trong y học
3. Overview of the application of artificial intelligence to gastrointestinal endoscopy Due to the rapid progression and poor prognosis of esophageal cancer (EC), the early detection and diagnosis of esophageal cancer is of great value. significant in improving the patient's prognosis. However, early endoscopic detection of esophageal cancer, especially Barrett's dysplasia or esophageal squamous dysplasia, is difficult. Therefore, the demand for more effective methods of detecting early esophageal cancer features has led to intensive research in the field of artificial intelligence (AI). Deep learning (DL) has brought about breakthroughs in image, video and other aspects of processing, while complex neural networks (CNN) have paved the way for detect high-resolution endoscopic images and videos.
Many studies on complex neural networks in early esophageal cancer endoscopic image analysis show excellent performance including sensitivity and specificity and gradual progression from print image analysis in vitro for classification to real-time detection of esophageal tumors in practice. As AI techniques enter pathology, contour lesions that are difficult to identify by endoscopists may become easier than before. In genomics, since genomic markers lack tissue specificity, they can now only be used as complementary measures. In predicting cancer risk, there is still a lack of prospective clinical studies to confirm the accuracy of the risk stratification model.
4. Introduction to Esophageal Cancer Esophageal cancer (EC) is the eighth most common cancer and the sixth leading cause of cancer death worldwide. Esophageal cancers mainly include esophageal adenocarcinoma (EAC) and esophageal squamous cell carcinoma (ESCC). Esophageal adenocarcinoma is the most common pathology in Western countries, more than 40% of patients with esophageal adenocarcinoma are diagnosed after the disease has metastasized and the 5-year survival rate is less than 20. %.
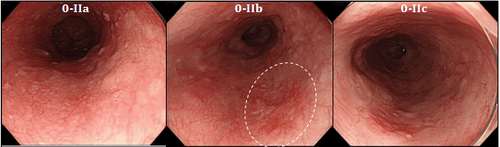
Hình 2: Ung thư thực quản giai đoạn sớm được nhìn thấy qua nội soi
Although the incidence of esophageal adenocarcinoma is increasing globally, esophageal squamous cell carcinoma remains the most common (80%) of all esophageal cancers with the highest incidence on the 'cancer belt' extending from East Africa and through the Middle East to Asia. Only 20% of patients with esophageal squamous cell carcinoma survive longer than 3 years, mainly due to late-stage diagnosis. In low-income areas, the 5-year survival rate is much lower at around 3.4%. Early diagnosis can significantly improve outcomes for all esophageal cancers.
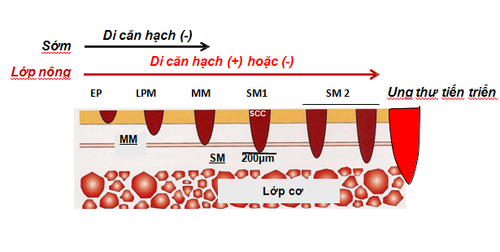
Hình 3: Phân chia giai đoạn của ung thư sớm thực quản
5. Role of AI in esophageal cancer detection 5.1 Barrett's dysplasia and early esophageal adenocarcinoma AI AI based on white light endoscopy (WLE) ) and NBI narrow-band endoscopic system: There are some limitations to the recognition of early cancerous lesions associated with Barrett's esophagus by WLE, a conventional technology. High-definition WLE (HD-WLE) and the NBI endoscopy system were once considered to improve the accuracy of diagnosing early cancerous lesions associated with Barrett's esophagus. However, the improvement has not yet satisfied the endoscopists. This situation stimulated the development of a CAD system for early cancerous lesions in Barrett's esophagus based on a supervised ML blood learning algorithm. In addition, this system still has difficulty in identifying early cancerous lesions associated with Barrett's esophagus and selecting biopsy sites.
To solve those problems, Ebigbo et al established a CAD system based on deep learning algorithms. The accuracy of the system using HD-WLE is better than that of the general endoscope system. Furthermore, the system shows the ability to locate lesions and the regional matching rate between lesions by the system and local experts up to 72%. In general, the specificity of NBI is higher than that of conventional WLE. Compared to using HD-WLE, the system has no obvious advantage when using NBI system. However, there is a common problem in the above studies when the same image dataset was used in both the training phase and the validation phase. This obviously cannot reflect clinical practice, so it is very important to use different imaging datasets for training and validation.
De Groof et al used different HD-WLE images for training and testing, the results showed that the sensitivity and specificity of the system was significantly higher than that of general endoscopists . The combination of AI and HD-WLE/NBI, widely used in the clinic, performs well in the diagnosis of early BE-associated tumor lesions and outperforms the general endoscopes. . However, in different studies, the accuracy of the CAD system in lesion delineation and the criteria for assessing the ability to locate the lesion are quite conflicting. More high-quality studies are needed in the future.
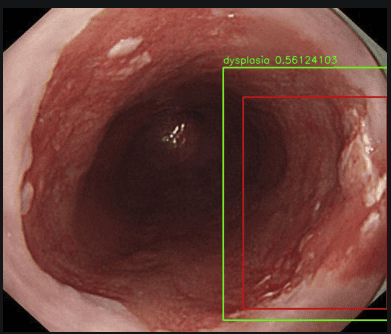
Hình 4: Hình ảnh loạn sản thực quản được đánh giá qua hệ thống nội soi có sử dụng trí tuệ nhân tạo AI, máy tính sẽ tìm khu trú tổn thương tự động (ô màu đỏ), đánh giá tổn thương đó có bao nhiêu phần trăm là ung thư
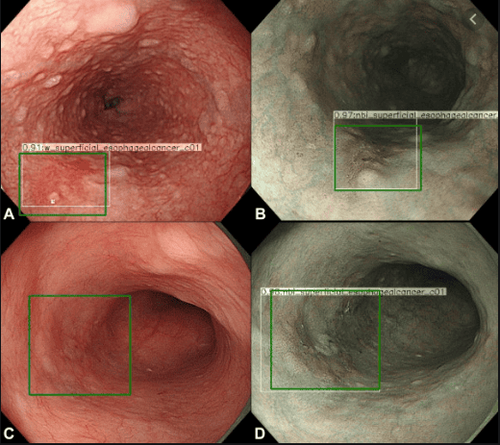
Hình 5: Sử dụng AI trong hệ thống nội soi: Ô vuông màu xanh là máy tính tự động khu trú hình ảnh tổn thương khi đưa máy soi đến.
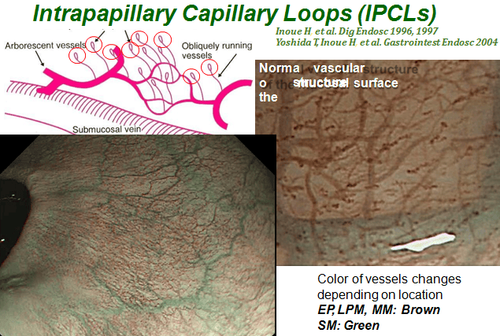
Hình 6: Hình ảnh rằng cấu trúc quai mao mạch IPCL được nhìn thấy trên nội soi NBI
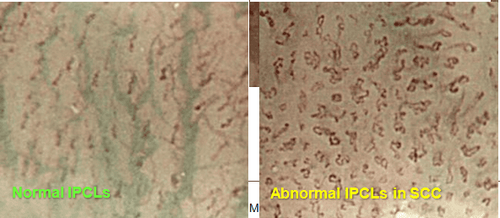
Hình 7: Hình ảnh IPCL bình thường và bất thường
6. The role of AI in genetic diagnosis of early esophageal cancer As mentioned above, the invasiveness of endoscopic diagnosis and the variability of pathological diagnosis, together with the advances Recent sets in the pathogenesis of esophageal cancer and the development of different omics technologies have made early EC gene diagnosis a hot research topic. Zhang et al and Yu et al used microRNAs and long noncoding RNAs specifically expressed in patients with esophageal squamous cell carcinoma to establish diagnostic models, and the results showed that both can be used to effectively differentiate early from advanced tumours. Xing et al selected specific secretory proteins based on RNA transcriptome data of esophageal squamous cell carcinoma patients to establish a diagnostic model with high sensitivity but unsatisfactory specificity. for early esophageal squamous cell carcinoma.
It is possible that the selection of secretory proteins featured in this study was based on up-regulatory genes in tumor tissues and underutilized down-regulatory genes. In addition, Shen et al and Zhai et al used microRNA markers and proteins expressed specifically in the plasma of esophageal squamous cell carcinoma patients to build diagnostic and diagnostic models. found that both can effectively differentiate esophageal squamous dysplasia (ESD) from normal esophagus. However, compared with early esophageal squamous cell carcinoma, AI-assisted gene diagnosis of BE-associated dysplasia and early esophageal cancer has been less studied.
The results of Slaby et al. show that specific microRNAs in BE tissue can be used to distinguish BE with or without dysplasia. These markers may not be specific to esophageal cancer, so genetic diagnosis can only be used as an adjunct to endoscopy and pathology until specific markers of genetic diagnosis of esophageal cancer was established.
7. The role of AI in risk stratification of early esophageal cancer It is estimated that only 0.12% -0.43% of Barrett's esophagus patients can progress to early esophageal cancer, especially There is a special need to establish an effective model to predict the annual risk of early esophageal cancer in patients with Barrett's esophagus. Previously risk stratification was based primarily on the presence of dysplasia, but the effect was not inherently ideal. Critchley-Thorne et al established a predictive model based on characteristic differences in immunofluorescence markers and histopathology between Barrett's esophagus patients who developed early esophageal cancer. and those who do not have the disease. Results have not been encouraging, with more than 30% of Barrett's esophagus patients developing early esophageal cancer each year classified as low-risk.
Li et al established a prediction model based on single nucleotide polymorphism differences in the biopsy tissues of Barrett's esophagus patients with good performance to predict early esophageal cancer each year.
In conclusion, AI artificial intelligence is trying to be used for endoscopic detection, pathological diagnosis, genetic diagnosis and cancer risk prediction of early esophageal cancer. This is useful for endoscopists and pathologists to improve diagnostic accuracy and assist physicians in treatment and follow-up strategies.
Undeniably, AI artificial intelligence is very useful for endoscopists and pathologists to improve diagnostic accuracy to aid in treatment and provide follow-up strategies with the goal of The ultimate goal is to improve the patient's quality of life.
Actively screening for gastrointestinal cancer is the best and scientific method for early detection of gastrointestinal cancers, including common cancers such as esophageal cancer, stomach cancer, and gastric cancer. colon cancer. Vinmec International General Hospital currently has a package of screening and early detection of gastrointestinal cancer that combines clinical and paraclinical examination to bring about the most accurate results possible.
In addition to being performed by a team of experts and experienced doctors, patients will receive a gastroscopy, colonoscopy with an NBI endoscope with anesthesia. Peripheral blood cell total analysis (by laser counter). Automated prothrombin time test. Activated Partial Thromboplastin Time (APTT) test using an automated machine. General abdominal ultrasound... The application of technology and modern equipment to the examination will give accurate results, make the treatment more effective, and shorten the hospital stay for the patient. core.
Please dial HOTLINE for more information or register for an appointment HERE. Download MyVinmec app to make appointments faster and to manage your bookings easily.
References
1. Bray F, Ferlay J, Soerjomataram I, Siegel RL, Torre LA, Jemal A. Global cancer statistics 2018: GLOBOCAN estimates of incidence and mortality worldwide for 36 cancers in 185 countries. CA Cancer J Clin. 2018;68:394-424. [PubMed] [DOI]
2. Hur C, Miller M, Kong CY, Dowling EC, Nattinger KJ, Dunn M, Feuer EJ. Trends in esophageal adenocarcinoma incidence and mortality. Cancer. 2013;119:1149-1158. [PubMed] [DOI]
3. Thrift AP. The epidemic of oesophageal carcinoma: Where are we now? Cancer Epidemiol. 2016;41:88-95. [PubMed] [DOI]
4. Lu-Ming Huang, Wen-Juan Yang, Zhi-Yin Huang, Cheng-Wei Tang, Jing Li, Artificial intelligence technique in detection of early esophageal cancer, World J Gastroenterol. Oct 21, 2020; 26(39): 5959-5969