This is an automatically translated article.
Post by Master, Doctor Mai Vien Phuong - Department of Examination & Internal Medicine - Vinmec Central Park International General Hospital
Artificial intelligence (AI), especially deep learning modeling, is a promising innovation in colonoscopy. Recent studies have shown that AI is not only accurate in detecting colorectal polyps, but can also reduce missed rates. However, the application of AI in real-time detection has been hindered by the heterogeneity of AI models and study design as well as the lack of lasting results.
1. Artificial intelligence system used in the detection of colorectal polyps
Artificial intelligence (AI) has been applied in the medical field since the early 1950s. AI is defined as any machine with cognitive functions that mimic humans, such as problem solving or learning. practice. Machine learning models, which are a subtype of AI, are characterized by a set of methods that can automatically detect patterns in data and then use undetected patterns to predict outcomes. Conventional AI systems use a type of supervised machine learning model to extract the covariates of the training data to achieve pattern recognition or classification. It is important to note that each piece of information that is fed into the patient representation is called a covariate, and traditional machine learning, e.g., logistic regression, only examines the relationships of " predetermined event" with the outcome. However, the machine learning model cannot change the way covariates are determined. Deep learning models really solve this problem by identifying covariates and building complex concepts from simple covariates, which are particularly useful in image classification and object placement because of The characteristics of a group of similar objects can be complex and difficult to define by humans (Figure 1).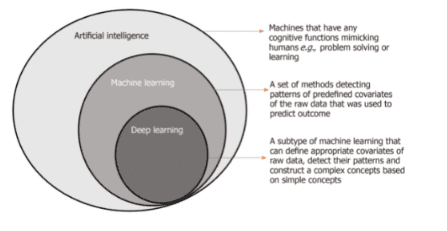
Hình 1. Trình bày sơ đồ về trí tuệ nhân tạo, máy học và học sâu.
2. The artificial intelligence system has the ability to remind the doctor about the time and speed to withdraw the bronchoscope to avoid missing polyps
In addition to the polyp locator system, Gong et al. reported a CADe system intended to monitor the rate of bronchoscope retraction in real time and to minimize blind spots during withdrawal. Their study showed that ADR was also improved from 8% to 16% (P = 0.001) with the CADe system. Similarly, Su et al. reported an automated quality control system for the detection of colorectal polyps and adenomas that would also remind the endoscopist of the timing and rate of retraction and the need for colonoscopy. Indistinct colon segments should be rechecked on the polyp locator system. Systemic was found to have a significantly higher ADR than conventional colonoscopy (28.9% vs 16.5%, P < 0.001).
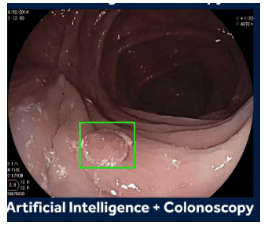
Phát hiện polyp đại tràng bằng AI
3. AI's role in missed polyps
In addition to its role in enhancing colorectal polyp detection, there are emerging data that suggest AI may also help minimize lesions missed during colonoscopy. In the authors' recent study, the authors showed that a validated real-time deep learning AI model can help endoscopists prevent missed colorectal lesions. First, the authors applied a validated AI system to review 65 videos of parallel examinations of the proximal colon (from cecum to splenic flexure) and found that the AI system could detect Up to 79.1% of adenomas were missed by the endoscopist during the first labor. test. In the second part of the prospective study, the same deep learning AI model was able to detect missed adenomas in 26.9% of patients during real-time screening. In multivariable analysis, missed adenoma was associated with finding multiple polyps during colonoscopy (adjusted OR, 1.05) or colonoscopy performed by less experienced endoscopes ( Corrected OR, 1.30).
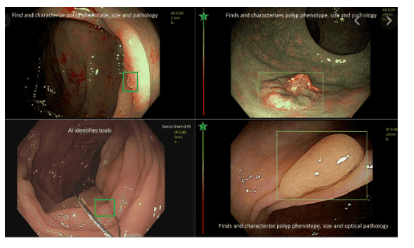
Trí tuệ nhân tạo giúp tăng tỉ lệ phát hiện polyp cho các bác sĩ
A recent single-center randomized controlled study by Wang et al also showed that the use of CADe-assisted colonoscopy was able to reduce AMR from 40.0% to 14.0% . In particular, there have been significant improvements in the ascending, traversing, and descending colon. However, the AMR in this randomized controlled study (up to 40%) was much higher than previously reported. Therefore, a multicenter trial will still be required to confirm this result. AI can act as an additional 'eye' for endoscopists While supporting AI's role in reducing missed lesions, these results suggest a key reason for adenomas omissions can still be due to human factors, as almost 80% of these missed lesions are actually displayed on the screen and are not detected by the endoscopist for various reasons, such as inexperienced, tired or distracted. Thus, AI can act as an additional “eye” for the endoscopist where distraction and fatigue never occur.
However, the authors' study also shows that about 20% of missed adenomas remain undetected by AI. These missed lesions are usually not visible “on screen”. They are located behind a fold or in a difficult to bend position or hidden under the stool in patients with poor bowel preparation. In a recent meta-analysis by Zhao et al that included 43 studies and 15000 parallel colonoscopy, the use of ancillary techniques and good bowel preparation was associated with fewer missed adenomas. . Intuitively, the combination of AI and ancillary devices with satisfactory bowel preparation may be necessary to completely eliminate the risk of missed colonoscopy during colonoscopy. Using artificial intelligence in histopathological evaluation of polyps
In addition to detecting colorectal polyps, AI has also been shown to be accurate in histological prediction and identification of polyps in a single patient. number of studies. Despite a high degree of heterogeneity in algorithms and designs, along with potential selection biases, studies that use deep learning models as a basis often perform better than those that do not. use other types of algorithms. A study by Byrne et al. has shown that using deep learning models can achieve 94% accuracy in real-time polyp classification. A similar result was reproduced by one study using magnified colonoscopy and both studies using narrowband imaging as the imaging technique. The authors' recent meta-analysis further showed that the composite accuracy from narrowband imaging studies was generally better than non-narrowband imaging studies in histological characteristics.
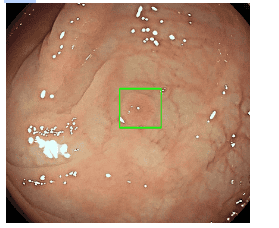
AI giúp đánh giá bản chất mô học polyp với độ chính xác cao
4. Limitations and directions for the future
Although there are already promising potential trials supporting the use of AI in real-time polyp detection during colonoscopy, there are several issues that need to be addressed before AI can be implemented in routine clinical practice. Because AI algorithms and deep learning models are still evolving and there is significant heterogeneity between different models and training data, an independent potential validation will be required for each model. AI system. The latest guidelines issued by the European Society of Gastroenterology suggest that the ability to incorporate computer-aided diagnosis (detection and characterization of lesions) on colonoscopy should supported by acceptable and reproducible accuracy for colorectal cancer, as demonstrated in high-quality multicenter clinical studies. Another important question regarding the use of AI in colonoscopy is the actual impact on long-term clinical outcomes. It remains unclear whether the use of AI-assisted colonoscopy can reduce the incidence of PCCRC or prolong the currently recommended follow-up interval after colonoscopy, which is will need long-term prospective cohort studies to resolve. AI's current role in colonoscopy could act as a virtual assistant for the endoscopist during real-time colonoscopy virtual assistant for endoscopist during real-time colonoscopy, especially in monitoring withdrawal time and polyp detection. The prospect of a fully automated stand-alone colonoscopy system is still too early at this stage. Furthermore, the “black box” nature of AI algorithms, especially deep learning models, may require considerable effort to convince regulators to approve them for regular use. Liability and compensation issues related to AI system manufacturers also need to be addressed. Therefore, there are still significant obstacles to overcome before the adoption of AI-assisted colonoscopy becomes widespread in everyday practice.
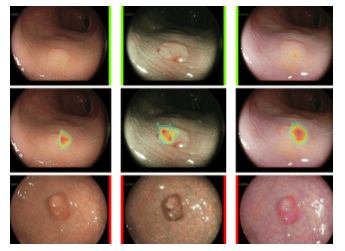
Nhiều thuật toán AI được sủ dụng trong nội soi giúp đánh giá polyp chính xác hơn
Conclusion
Externally validated AI system can be one of the promising solutions to increase the detection of adenomas and minimize the missed lesions during real-time colonoscopy . However, means to ensure adequate mucosal contact, such as complementary devices and optimal bowel preparation, are also important in reducing polyp missed rates in colonoscopy practice. colon daily. Long-term data are also needed to determine the actual clinical benefit of this emerging technology in reducing PCCRC.
Please dial HOTLINE for more information or register for an appointment HERE. Download MyVinmec app to make appointments faster and to manage your bookings easily.
References Lui TKL, Leung WK. Is artificial intelligence the final answer to missed polyps in colonoscopy? World J Gastroenterol 2020; 26(35): 5248-5255 [PMID: 32994685 DOI: 10.3748/wjg.v26.i35.5248]