This is an automatically translated article.
Post by Master, Doctor Mai Vien Phuong - Department of Examination & Internal Medicine - Vinmec Central Park International General Hospital
The recognition mechanism of artificial intelligence (AI) is an interesting topic in understanding artificial intelligence neural networks and their application in treatment. Some layered neural networks can recognize cancer through deep learning algorithms. It will be interesting to think about whether human insights and artificial intelligence attention are related, which is one of the main points in this article.
Automated cancer detection with computer-aided diagnosis is being implemented in the clinic and will be improved with feature mapping in neural networks. Cancer subtypes and stages, in terms of progression and metastasis, should be graded with artificial intelligence for optimal treatment.
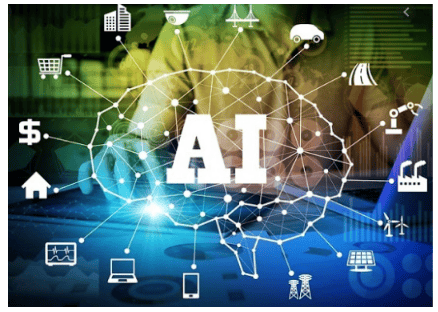
1. Overview
Automated cancer detection has been implemented and will become more common. Computer-aided diagnosis (CAD) is evolving, and cancer detection and classification has been achieved in identifying subtypes of leukemia with dense convolutional and neural networks. -ron complex. A computer-aided diagnostic system with a giant artificial neural network based on soft tissue techniques has detected lung cancer in X-ray images. Helicobacter pylori infection was predicted by endoscopic imaging using artificial intelligence. A faster region-based convolutional neural network was applied to diagnose T stage of gastric cancer in advanced computed tomography (CT) imaging of gastric cancer. Digital imaging of pathological data in oncology has been used in cancer diagnosis. Digital pathology analysis using whole-slice imaging can contribute to the “remote” assessment. Automated image analysis and increasing artificial intelligence applications in the field of thyroid pathology. Cancer recognition by artificial intelligence has become more accurate and precise, accompanied by the advancement of neural networks and computational capabilities.
2. Recognizing and applying artificial intelligence
It is possible that deep learning algorithmic approaches such as biomedical text mining models pre-trained in natural language corpus can be applied to cancer recognition by artificial intelligence. The recognition mechanism of the artificial intelligence application can be translated into human language through the sign of attention. Future perspectives on cancer identification in AI may need to focus on AI translation and human language. Survival of liver cancer patients can be predicted with multi-element integration based on deep learning algorithm. The Autoencoder architecture was used to integrate RNA sequencing data (RNA-Seq), DNA methylation data and microRNA sequencing data (miRNA-Seq) of hepatocellular carcinoma in the database. cancer gene atlas (TCGA) data.
Data coordination with TCGA-Assembler is the first step to providing appropriate data for artificial intelligence.
A homology network fusion approach predicted cancer subtypes and survival. Gene markers for metastatic-associated recurrence of HCC were identified using a classification model that included class prediction algorithms, support vector machines (SVMs), proximal centroids, 3 nearest neighbors, 1 nearest neighbor, linear discriminant analysis and predictive covariates, to assess the risk of cancer recurrence in the early stages. Gene sets of mutations have been identified in liver cancer, including hepatitis-positive samples. Learning SVM is very useful for cancer staging and classification. Tumor pathology, such as grading, grading and staging, can be predicted by artificial intelligence based on deep learning algorithms. Clustering and machine learning methods were used to classify breast cancer patients with immunotherapy. Advanced non-muscle-invasive bladder cancer and muscle-invasive bladder cancer are classified based on the molecular subtype of responsiveness to immunotherapy. An interesting classification model called unknown primary cancer-artificial intelligence-Dx predicted tumor primary location and molecular subtype in RNA profiles.
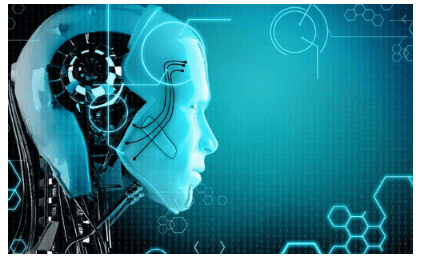
3. Application of artificial intelligence technology in cancer treatment
Enhanced clinical workflow with proposed AI interventions in cancer treatment, including AI-guided detection and characterization, planning and monitoring artificial intelligence-guided treatment and artificial intelligence-driven outcome optimization. Artificial intelligence tools can be used to detect abnormalities, characterize suspected lesions, and determine prognosis or response to treatment. Artificial intelligence technology provides powerful tumor descriptors in segmentation, diagnosis, staging, and genomic imaging. Radiographic characterization from CT Scan images of lung cancer patients was successful to show an association with gene expression and prognostic potential. CT-based radiographic features can predict distant metastasis for lung adenocarcinoma patients.
A new approach in the evaluation and validation of biomarkers that brings the revised criteria in imaging data into the Criteria for Evaluation of Solid Tumor Response in Cancer Therapy. Results of a clinical study in metastatic non-small cell lung cancer demonstrated that pembrolizumab combined with chemotherapy resulted in a longer overall survival and progression-free survival compared with chemotherapy alone. in patients with no epidermal growth factor receptor or non-producing lymphoma kinase mutations. The application of artificial intelligence in medical fields such as early detection, diagnosis and treatment of diseases is expanding. Clinical data is processed by natural language processing and machine learning of artificial intelligence, which will be important components in making clinical decisions about treatment strategies
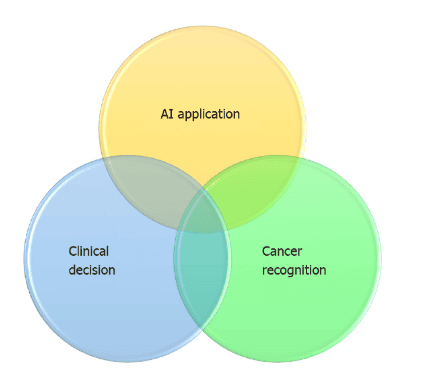
Application of artificial intelligence in cancer recognition and treatment.
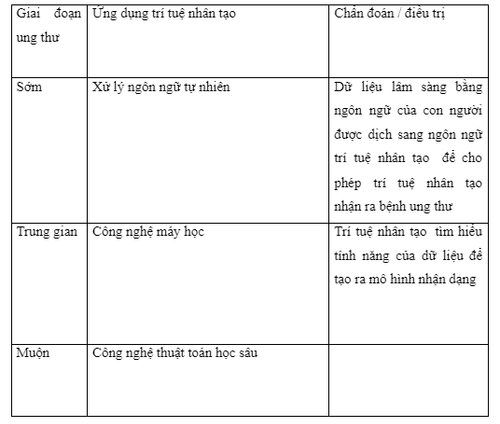
Conclusion
The use of artificial intelligence for cancer identification is increasing rapidly. The traditional approach can evolve with neural networks of artificial intelligence to create a future field for the planet. The recognition of image data, as well as translated and untranslated copies of genes in cancer, will deepen the field of artificial intelligence.
Please dial HOTLINE for more information or register for an appointment HERE. Download MyVinmec app to make appointments faster and to manage your bookings easily.
References
Tanabe S. Cancer recognition of artificial intelligence. Artif Intell Cancer 2021; 2(1): 1-6 [DOI: 10.35713/aic.v2.i1.1]