This is an automatically translated article.
Posted by Master, Doctor Mai Vien Phuong - Gastrointestinal Endoscopy - Department of Medical Examination & Internal Medicine - Vinmec Central Park International HospitalDue to the rapid progression and poor prognosis, the early detection and diagnosis of esophageal cancer is of great value in improving the patient's prognosis. Currently, science has applied artificial intelligence techniques in endoscopic combined optical tomography (EOCT) and endoscopic confocal laser (CLE) to diagnose esophageal cancer.
Abbreviation:
EC: Esophageal cancer
AI: artificial intelligence
BE: Barrett esophagus
1.What is Artificial Intelligence? Artificial Intelligence (AI: Artificial Intelligence) can be defined as a branch of computer science that deals with the automation of intelligent behaviors. AI is a part of computer science and therefore it must be based on solid, applicable theoretical principles of the field.
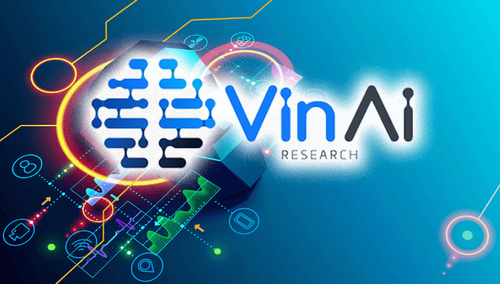
2. Overview of the application of artificial intelligence to gastrointestinal endoscopy
Due to the rapid progression and poor prognosis of esophageal cancer (EC), the early detection and diagnosis of esophageal cancer is of great value in improving the prognosis for patients. However, early endoscopic detection of esophageal cancer, especially Barrett's dysplasia or esophageal squamous dysplasia, is difficult. Therefore, the demand for more effective methods of detecting early esophageal cancer features has led to intensive research in the field of artificial intelligence (AI). Deep learning and memory techniques (DL) have brought about breakthroughs in image, video and other aspects of processing, while complex neural networks (CNN) have paved the way for detect high-resolution endoscopic images and videos.
3. Introduction to Esophageal Cancer Esophageal cancer (EC) is the eighth most common cancer and the sixth leading cause of cancer death worldwide. Esophageal cancers mainly include esophageal adenocarcinoma (EAC) and esophageal squamous cell carcinoma (ESCC). Esophageal adenocarcinoma is the most common disease in Western countries, more than 40% of patients with esophageal adenocarcinoma are diagnosed after the disease has metastasized, and the 5-year survival rate is less than 5 years. 20%.
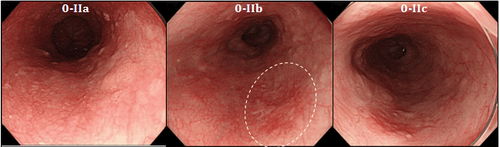
Although the incidence of esophageal adenocarcinoma is increasing globally, esophageal squamous cell carcinoma remains the most common (80%) of all esophageal cancers with the highest incidence on the 'cancer belt' extending from East Africa and through the Middle East to Asia. Only 20% of patients with esophageal squamous cell carcinoma survive longer than 3 years, mainly due to late-stage diagnosis. In low-resource areas, the 5-year survival rate is much lower at around 3.4%. Early diagnosis can significantly improve outcomes for all esophageal cancers.
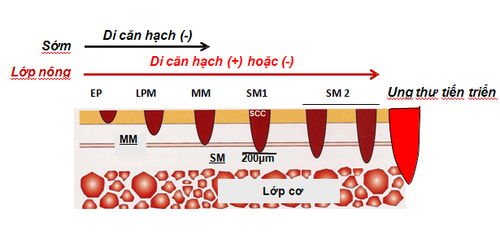
4. Application of Machine learning and Deep learning algorithms in esophageal cancer The demand for more effective early detection and characterization methods of esophageal cancer has led to in-depth research in the field artificial intelligence (AI), which can be identified by machine-established intelligence as opposed to the natural intelligence displayed by humans and other animals. Machine learning (ML) and deep learning (DL) are important parts of AI. Machine learning can be divided into supervised and unsupervised methods. Unsupervised learning is to identify groups in data according to commonalities, lacking knowledge of the number of groups or their meanings. When the training package contains input-output pairs, a supervised learning model is required to map the new input to the output. Conventional ML techniques are limited in their ability to process natural data in its raw form. In the early stages of research and development, model training was mainly with ML, through which researchers had to manually extract probable disease features based on clinical knowledge. The power of this computer-aided diagnosis (CAD) system is weak and insufficient to be applied in clinical real-time diagnosis.
5. The Role of a Convolutional Neural Network (CNN) A Convolutional Neural Network (CNN) is a supervised machine learning model inspired by the visual cortex of the human brain that processes and recognizes images . Each artificial neuron is a computational unit and all of them are interconnected, forming a network. Using multiple network layers, CNN can extract key features from an image with minimal pre-processing and then provide final classification through fully connected layers as output. The increasing competition for performance has led to the increasing complexity of pooling layers which led to the concept of deep learning. The important aspect of deep learning is that these feature classes are not designed by human engineers. They are learned from data using a general-purpose learning process. Deep learning has brought breakthroughs in image, video and other aspects of processing, while iterative CNNs have shed light on the detection of endoscopic images and videos.
6. The role of artificial intelligence AI in early detection of esophageal cancer 6.1. Barrett's dysplasia and early-stage esophageal adenocarcinoma Artificial intelligence based on white light endoscopy (WLE) and NBI narrowband endoscopy system. There are some limitations to recognizing early cancerous lesions associated with Barrett's esophagus by WLE, a common technology. High-definition WLE (HD-WLE) and the NBI endoscopy system were once considered to improve the accuracy of the diagnosis of early cancerous lesions associated with Barrett's esophagus.
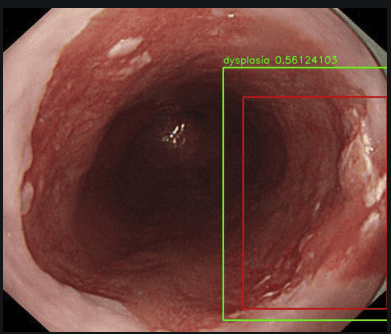
6.2. Role of endoscopic coherent optical tomography (EOCT) and endoscopic confocal laser (CLE) AI Artificial Intelligence based on endoscopic optical tomography and confocal laser endoscopy: Beyond WLE and NBI, endoscopic coherent optical tomography (EOCT) and endoscopic confocal laser (CLE) techniques are also used for early diagnosis of EAC/BE-associated dysplasia. EOCT can identify early tumor lesions associated with Barrett's esophagus by analyzing mucosal and submucosal structures of the esophagus. CLE can observe mucosal tissue and cell morphology to achieve optical biopsies. However, the complexity of these two imaging technologies, the time-consuming reading of the images, and the requirement of a senior endoscopist have limited their clinical use.
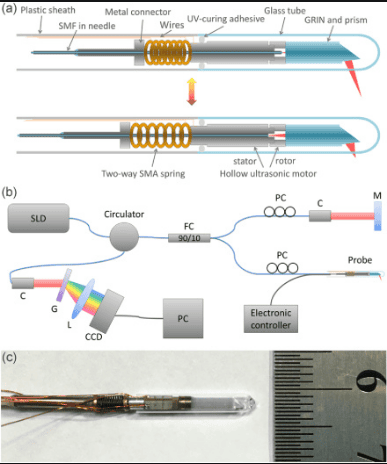
To solve this problem, Qi et al extracted multiple EOCT imaging features and combined one or more features to classify lesions, but the results were not satisfactory. Then, Swager et al used volumetric laser endoscopic imaging (VLE, integrated with second-generation OCT) for training and testing, and the results showed that the CAD system outperformed other models. VLE experts. Veronese et al used CLE imaging, and the results showed that the system was able to accurately differentiate gastric (GM), intestinal (IM) and neoplasia.
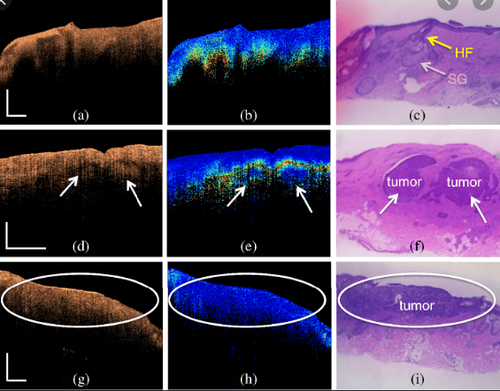
Ghatwary et al showed that the sensitivity of the system using CLE imaging to diagnose IM and cancer is significantly higher than that of the system for diagnosing GM. Similarly, the CAD system established by Hong did not identify the GM, the diagnostic sensitivity of IM was not significantly different from the above study, and the sensitivity for cancer diagnosis was also slightly reduced. This may be due to the limited number of GM and neoplastic images. Currently, there is little clinical research in this area and the available images are limited. In the future, more studies are needed to confirm the value of VLE/CLE combined with AI in early diagnosis of EAC.
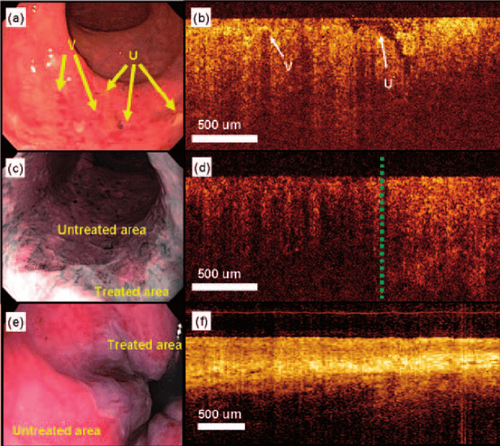
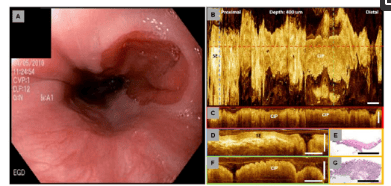
7. Real-time diagnosis using AI Currently, research in this area is limited. Ebigbo et al further optimized the CAD system based on previous research and applied it to clinical real-time detection for the first time. While 14 Barrett cancer patients underwent endoscopic examination, 62 endoscopic images (36 early esophageal carcinomas and 26 non-dysplastic Barrett's esophagus) were taken with the CAD system for real-time classification. , and the results showed sensitivity and specificity of 83.7% and 100%, respectively. There were no significant differences between the system and the experienced endoscopists. However, there are still some shortcomings in this study. First, the number of patients and images is low. Second, the system still uses images for diagnosis, not video for real-time detection. Finally, AI's ability to aid in lesion delineation and biopsy guidance remains unproven. In addition, the CAD system built by Hashimoto et al. can meet clinical real-time detection needs. Unfortunately, the researchers did not verify the system's performance in real-time diagnostics.
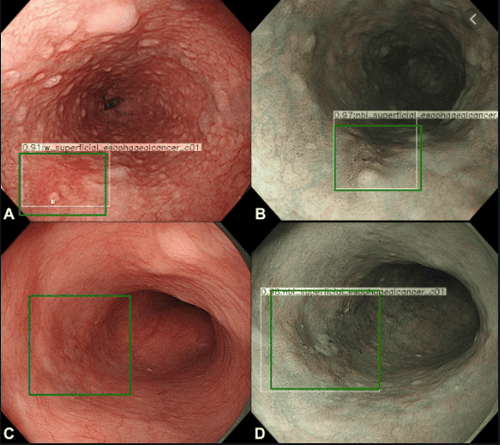
Tokai et al also used unmagnified endoscopic images for analysis and found that the sensitivity of NBI is slightly higher than that of WLE, and the diagnostic performance of the system is better than that of internal experts. soi. Further analysis showed that the accuracy of the CAD system in diagnosing tumors invasive to epithelial, choroidal, and muscular layers EP/LPM and MM is more than 90%, significantly higher than invasive tumor diagnosis. invades the submucosal layer SM1 and SM2. The reason may be that the training image set did not contain a normal esophageal image and the system confused extra-frame compression, failure to fully expand the esophagus wall, with the lesion features.
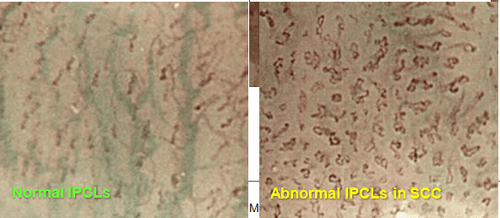
8. The role of artificial intelligence AI in endoscopy (endoscopy at the cellular level)
AI artificial intelligence based on high resolution endoscopy and micro endoscopy: Endoscopic endoscopy (endoscopy at the cellular level) is a new technology that combines magnified endoscopy with important dye. Due to the excellent magnification, the endoscope can clearly visualize the epithelial cells of the esophageal mucosa to achieve an effect similar to the diagnosis of pathology.
However, if endoscopists want to use endoscopes to independently complete real-time detection, they need a strong background in pathology, which is clearly impractical. Therefore, AI may be the best choice to aid in endoscopic diagnosis. Kumagai et al. have shown that the performance of the system using higher magnification images is better than the system using lower magnification images. However, there was no stratification analysis of superficial and advanced esophageal cancer in this study.
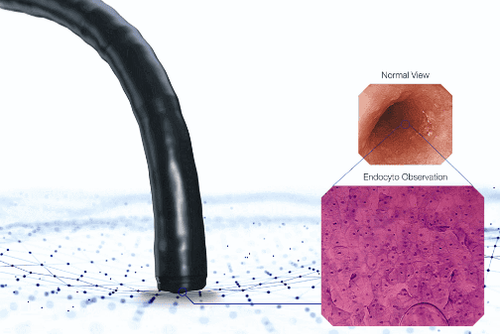
In conclusion, AI artificial intelligence is trying to be used for endoscopic detection, pathological diagnosis, genetic diagnosis and cancer risk prediction of early esophageal cancer. It is useful for endoscopists and pathologists to improve diagnostic accuracy and assist physicians in treatment and follow-up strategies. Boundary lesions of esophageal cancer pathology are still difficult to define which could be the main direction of AI-assisted pathology diagnosis in the future.
Currently, screening for gastrointestinal cancer is a scientific and effective measure for early detection of gastrointestinal cancer (esophageal cancer, stomach cancer, colon cancer) and giving a treatment plan. best treatment. Currently, Vinmec International General Hospital has a package of screening and early detection of cancers of the gastrointestinal tract (esophagus - stomach - colon) combined with clinical and paraclinical examination to bring the most accurate results. maybe.
When screening for gastrointestinal cancer at Vinmec, you will receive:
Gastrointestinal specialty examination with an oncologist (by appointment). Gastroscopy and colonoscopy with an NBI endoscope with anesthesia. Peripheral blood count (laser counter). Automated prothrombin time test. Automated thrombin time test. Activated Partial Thromboplastin Time (APTT) test using an automated machine. General abdominal ultrasound
Please dial HOTLINE for more information or register for an appointment HERE. Download MyVinmec app to make appointments faster and to manage your bookings easily.
References
Bray F , Ferlay J, Soerjomataram I, Siegel RL, Torre LA, Jemal A. Global cancer statistics 2018: GLOBOCAN estimates of incidence and mortality worldwide for 36 cancers in 185 countries. CA Cancer J Clin . 2018; 68 :394-424. [PubMed] [DOI] Hur C, Miller M, Kong CY, Dowling EC, Nattinger KJ, Dunn M, Feuer EJ. Trends in esophageal adenocarcinoma incidence and mortality. Cancer . two thousand and thirteen; 119 :1149-1158. [PubMed] [DOI] Thrift AP . The epidemic of oesophageal carcinoma: Where are we now? Cancer Epidemiol . 2016; 41 :88-95. [PubMed] [DOI] Lu-Ming Huang, Wen-Juan Yang, Zhi-Yin Huang, Cheng-Wei Tang, Jing Li, Artificial intelligence technique in detection of early esophageal cancer, World J Gastroenterol. Oct 21, 2020; 26(39): 5959-5969