This is an automatically translated article.
Posted by Master, Doctor Mai Vien Phuong - Gastrointestinal Endoscopy - Department of Medical Examination & Internal Medicine - Vinmec Central Park International General Hospital.Artificial neural networks (artificial neural networks) belong to a subtype of artificial intelligence and have been used in many subspecialties of clinical medicine, including pathology, radiology, Cardiology, neurology, orthopedics and gastroenterology. So what are the limitations and challenges of neural networks in the future of medicine?
1. Overview
The concept of artificial intelligence (AI) was first proposed at the Dartmouth Conference in 1956. Although definitions of artificial intelligence differ, it is universally accepted that artificial intelligence is designed designed to perform tasks that were previously only possible by the human mind. Artificial neural networks (artificial neural networks) belong to a subtype of artificial intelligence and have been used in many subspecialties of clinical medicine, including pathology, radiology, cardiology vascular, neurology, orthopedics and gastroenterology. Gastrointestinal disorders are diseases of the human digestive system and treatment regimens for digestive diseases rely heavily on imaging tests. Bulk imaging data is a burden on radiologists, potentially increasing the odds of making incorrect clinical decisions. Compelling evidence shows that artificial neural networks can effectively solve this problem.
2. Features of Artificial Neural Network
Accumulating studies have demonstrated that artificial neural networks can have significant potential in the diagnosis and treatment of digestive diseases. Models based on artificial neural networks often have optimal accuracy and AUC values. Some evaluation indicators of artificial neural network models, even reaching 100% accuracy. For further validation of the artificial neural network indices, comparisons were also performed and can be divided into three aspects based on the compared objects. First, regarding experts, studies involving comparisons with experts aim to show that artificial neural networks are superior to artificial diagnoses.
Most artificial neural networks show faster speed and better accuracy than professional clinicians. Therefore, some authors claim that artificial neural networks will become great tools for doctors and scientists, possibly even replacing humans in this capacity. Second, different artificial neural network models have been studied. The researchers used several artificial neural network algorithms that were trained with the same data set and went through validation stages. The models with the best fit are then singled out for processing for further exploration. The comparisons show the ability of different models to handle clinical problems. Finally, other reported data have shown that learning from textbooks is an alternative approach to determine the effectiveness of developed models.
However, this type of comparison method is unreliable due to inconsistencies of the study baselines, such as differences in data sets, hardware performance, and runtime. These unspecified deviations may also affect the comparison results. Therefore, this method is not recommended for further studies to further verify the advantages of the models. Collectively, compelling evidence indicates that artificial neural networks can lead the classification and in-depth analysis of gastrointestinal clinical practice compared with linear statistical models and human labor.
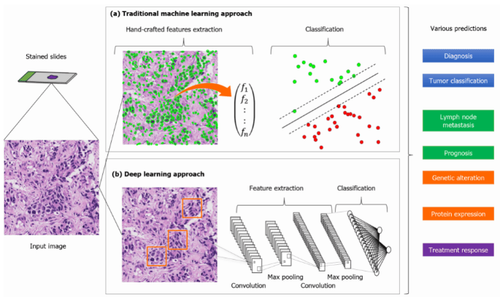
3. Cost is also an important factor for clinical practice
To train a qualified physician requires considerable social and economic time and costs. However, learning artificial neural networks is a relatively simple process. Based on current reports, training artificial neural network models with large sample databases only takes a few days. Artificial Neural Networks can comprehensively analyze data features and adjust weight values in extremely short time. Moreover, hardware is the basic requirement to run artificial neural network algorithms, especially the graphics card and central processing unit, two important data processing factors. High-performance hardware costs several thousand dollars, much cheaper than training a doctor. In general, the time and economic costs to train artificial neural network models are much smaller than training doctors. It is believed that artificial neural networks will play an important role in clinical practice in the near future.4. Computers outperform humans in data processing such as in medical research
A comprehensive diagnosis of digestive diseases requires a variety of tests. The gastroenterologist must integrate clinical indicators to make a diagnosis. However, existing guidelines and expert consensus cannot help gastroenterologists manage the majority of complex diseases. Artificial neural networks can mitigate this limitation. Various types of patient characteristics and clinical indicators can be included in predictive models. Data stored in images is geometrically higher than textual data. CNN can interpret X-ray and endoscopic images. CNN's analysis speed is even fast enough that real-time detection can be achieved. Several studies simultaneously included clinical indicators and imaging information to analyze the patient's situation.5. Limitations to consider of artificial neural networks
The application of artificial neural networks seems to be a way to overcome existing problems for gastroenterologists. However, certain limitations need to be seriously considered. First, the training method affects the performance of the neural network models. Most research groups have performed small sample studies with less than 1000 cases. For endoscopic detection, although the number of frozen frames can be as high as ten thousand, some frames are from the same video. As a result, the repeatability of frames reduces their representativeness. The small size of the dataset leads to a higher likelihood of overfitting, which refers to models that are overtrained and overfit the internal data set characteristics. Rigid observation of the internal data sets naturally reduces the flexibility of the model to manage the external data. For example, if the model is trained with a dataset of patients from country A, patients from country B may not be well described by this model due to different factors. Otherwise, the retrospective dataset should be used as the primary source of neural network training. Similar to conventional retrospective studies, biases also exist and affect the application of the models. Selection bias is the most important factor. Patient data from medical history may be selected based on research programs. The information of registered cases is not flexible, meaning that the researchers cannot rule out the possibility of bias as they would expect.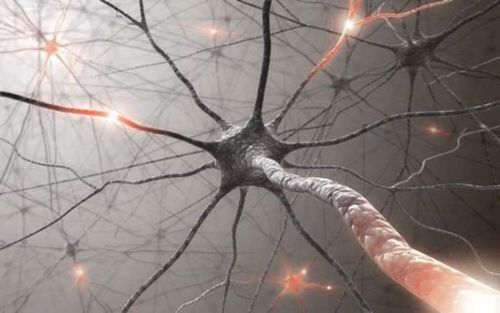
6. The trade-off between interpretability and accuracy should be emphasized
The second of the limitations is the trade-off between interpretability and accuracy that should be emphasized. Models with optimal accuracy often lack interpretability (i.e., interpretability). In contrast, models with near-perfect interpretation are less accurate, such as linear regression. During the training of the artificial neural network, the modulation of the algorithm and the data feedback is blinded. The researchers do not know the activation functions in the hidden layers, similar to a machine that hides the circuit in a box and puts only the switches and the display outside; This feature is called "black box". For important medical decisions, doctors and patients both have the right to know how decisions were made. Not being able to understand how the model makes decisions will naturally reduce the trust of patients and doctors. In contrast, lack of explanatory power makes it impossible for researchers to describe the inference processes of artificial neural network models, reducing their reference value.
7. Sociological issues need to be carefully considered for neural network applications
The third of the limitations of neural networks are sociological issues that need careful consideration for neural network applications, which can be divided into three aspects. First, regarding medical liability, medical errors and malpractice can occur, although the artificial neural network has an excellent track record in the validation phase. Thus, who is responsible for decisions and how to allocate liability for damages is difficult to determine. Doctors, patients, and programmers all seem to be innocent, and accidents are mostly caused by inherent shortcomings, not by human carelessness. Second, regarding security, the operation of artificial neural network models requires many types of data, some of which are related to patient privacy. Doctors who betray privacy will receive punishment. However, artificial neural network models are connected to the Internet or a company's intranet. In fact, it is difficult to discern the source of a privacy disclosure. Moreover, the data extraction capabilities of artificial neural networks are unmatched. The consent waivers therefore require further debate. The balance between neural network research and patient privacy remains a challenge.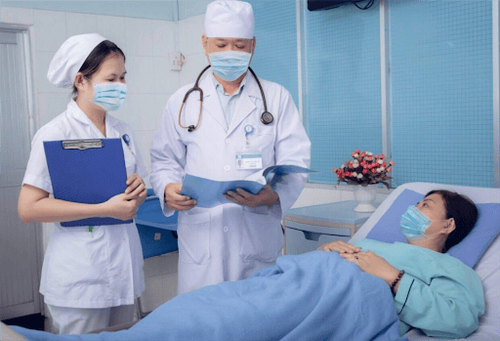
8. Multi-stakeholder participation is an important feature of artificial neural networks
Finally, in terms of legislation, multi-stakeholder participation is an important feature of artificial neural networks, including hospitals, patients, product providers, and insurance companies. Unfortunately, the rules for artificial neural networks used in medical practice are still poorly understood. Current legislation cannot support the application and prospective studies of artificial neural networks. Legally, multi-stakeholder engagement is an important feature of artificial neural networks, including hospitals, patients, product providers, and insurance companies. Unfortunately, the rules for artificial neural networks used in medical practice are still poorly understood. Existing legal regulations cannot support the application and prospective studies of artificial neural networks.
Multi-stakeholder participation is an important feature of artificial neural networks, including hospitals, patients, product providers, and insurance companies. Unfortunately, the rules for artificial neural networks used in medical practice are still poorly understood. Existing legal regulations cannot support the application and prospective studies of artificial neural networks.
Please dial HOTLINE for more information or register for an appointment HERE. Download MyVinmec app to make appointments faster and to manage your bookings easily.
References
Cao B, Zhang KC, Wei B, Chen L. Status quo and future prospects of artificial neural network from the perspective of gastroenterologists. World J Gastroenterol 2021; 27(21): 2681-2709 [DOI: 10.3748/wjg.v27.i21.2681]
Karakitsos P , Ioakim-Liossi A, Pouliakis A, Botsoli-Stergiou EM, Tzivras M, Archimanditis A, Kyrkou K. A study Comparative study of three variants of the vector quantifier in differentiating benign from malignant gastric cells. Cytology . In 1998; 9: 114-125. [ PubMed ] [Cited in This Article: 2]