This is an automatically translated article.
Post by Master, Doctor Mai Vien Phuong - Gastrointestinal Endoscopy - Department of Medical Examination & Internal Medicine - Vinmec Central Park International General Hospital.Duration of life and quality of life are important factors that both patients and physicians consider. Current studies have used artificial neural networks to consider a variety of factors to quantify survival outcomes for patients with cancer, gastrointestinal bleeding, and inflammatory bowel disease.
1. Overview of Artificial Neural Networks
Artificial neural networks (artificial neural networks) belong to a subtype of artificial intelligence and have been used in many sub-specialties of clinical medicine, including pathology, radiology, Cardiology, neurology, orthopedics and gastroenterology.
Gastrointestinal disorders are diseases of the digestive system and the treatment regimen is based on imaging tests. Bulk imaging data is a burden on radiologists, potentially increasing the rate of incorrect clinical decisions. Compelling evidence suggests that artificial neural networks can effectively solve this problem. In contrast, the rapid improvement of artificial neural networks requires clinicians to add more knowledge and fully understand the strengths and limitations of artificial neural networks.
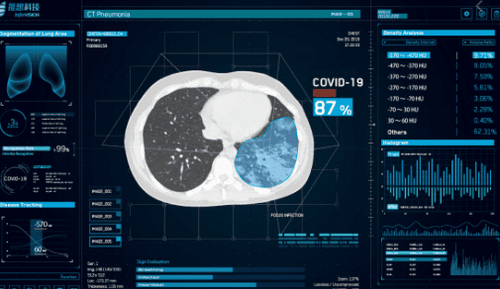
2. The role of artificial neural networks in predicting disease risk and patient survival prognosis
A research team conducted a retrospective study of 521 gastric cancer patients in 2005. Neural networks are one of three techniques used to analyze clinical information and predict variables. symptoms after surgery. In which, the artificial neural network does better than the other two methods. Since then, many studies have focused on the clinical potential of artificial neural networks in predicting disease risk as well as patient survival.
In particular, a large number of related reports have appeared in recent years, which is reflected in the rapid improvement of machine learning algorithms. Artificial neural network models for cancer risk prediction often include dozens of categorical variables, such as age, sex, cancer indices, radiological tests, and treatment regimens.
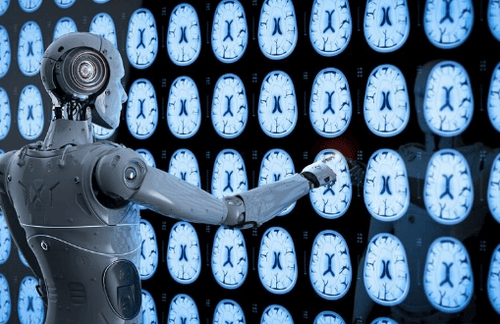
Two groups of Japanese people combined clinical indicators and endoscopic images using CNN to predict gastric cancer risk. Genetic and microbial factors function as important regulators of carcinogenesis. Genomics and microbiota may represent the underlying origins of gastrointestinal tumors.
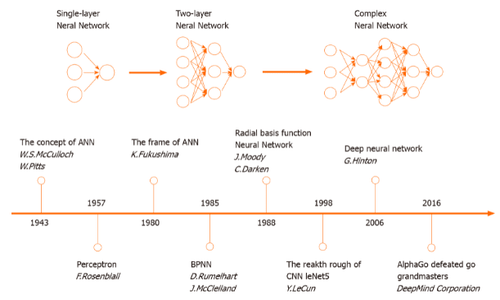
3. Advantages of artificial neural networks in diagnosing digestive cancer metastasis
Metastasis is an important indicator of long-term treatment and prognosis. CT and MRI have limitations in detection and can only observe positive lymph nodes with swelling, distant metastatic lesions. However, the results always lag behind the actual situation, leading to mistakes in surgical strategy and reducing the survival benefit for the patient.
Therefore, the advantages of artificial neural networks are being used to improve the diagnosis of metastatic conditions in gastrointestinal cancer. A group of Germans used FNN to predict lymph node metastasis of gastric cancer as early as 2005, but the performance was poor, in part because of unimproved algorithms and computers. With the development of artificial neural networks, models include larger numbers and more subtypes of clinical indicators. Their predictive efficacy has been enhanced to meet clinical criteria.
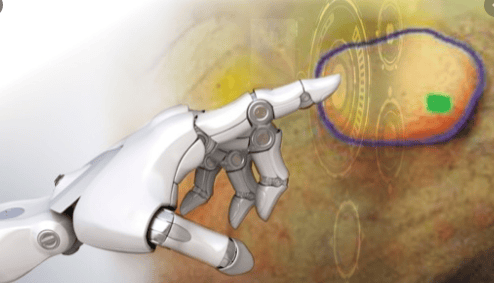
Two studies investigating artificial neural networks used to determine metastatic risk of patients with stage T1 colorectal cancer. Although cases with metastatic T1 tumors are rare, the underlying mechanisms can be explored with the help of artificial neural networks.
4. Artificial neural network predicts the survival of cancer patients
Duration of life and quality of life are important factors that both patients and physicians consider. To date, no effective approaches have been developed, which is often explained by disease heterogeneity, subpopulation differences, and medical experience. These problems may be based on a lack of data integration for one reason. Investigators pin their hopes on an accurate diagnosis based on the amazing ability of information screening and automated decision making. They used an artificial neural network to weigh a variety of factors to quantify the survival outcomes of patients with cancer, gastrointestinal bleeding, and inflammatory bowel disease. For gastrointestinal cancer, several studies have compared artificial neural networks with TNM staging and models built using other machine learning methods. Artificial Neural Networks often exhibit better performance than Logistic regression, TNM stage and even clinician. Competitions between types of artificial neural networks were also performed, and the results were inconsistent.
Please dial HOTLINE for more information or register for an appointment HERE. Download MyVinmec app to make appointments faster and to manage your bookings easily.
References:
Cao B, Zhang KC, Wei B, Chen L. Status quo and future prospects of artificial neural network from the perspective of gastroenterologists. World J Gastroenterol 2021; 27(21): 2681-2709 [DOI: 10.3748/wjg.v27.i21.2681]