This is an automatically translated article.
The article is written by MSc, BS. Mai Vien Phuong, Department of Medical Examination & Internal Medicine - Vinmec Central Park International General HospitalArtificial intelligence has the potential to be applied during colonoscopy to automatically detect colorectal polyps and distinguish between cancerous and noncancerous polyps, with the potential to improve detection rates. adenomas, the incidence varies widely among endoscopists when performing a colonoscopy examination. Currently, there are many methods of testing for H.pylori that are applied in clinical and research. Depending on whether the method requires gastroduodenal endoscopy or not, people are divided into two groups: invasive methods and non-invasive methods.
1. Overview
Artificial intelligence (AI) enables machines to provide disruptive value in a number of industries and applications. Applications of AI techniques, especially machine learning and more recently deep learning, are growing in gastroenterology. Computer-aided diagnosis of upper gastrointestinal endoscopy is increasingly attracting attention for the automatic and accurate identification of dysplasia in Barrett's esophagus, as well as for the early detection of gastric cancers (GCs). , thus preventing malignancies in the esophagus and stomach. In addition, sophisticated neural network technology can accurately assess Helicobacter pylori (H. pylori) infection during standard endoscopy without biopsy, thereby reducing the risk of cancer stomach letter. Artificial intelligence has the potential to be applied during colonoscopy to automatically detect colorectal polyps and distinguish between cancerous and noncancerous polyps, with the potential to improve detection rates. adenomas, the incidence varies widely among endoscopists when performing a colonoscopy examination. In addition, AI allows to establish the feasibility of endoscopic polypectomy of large colon polyp lesions based on surface features and microvascular changes.2. Introduction
Artificial intelligence (AI) is based on artificial intelligence elements that perform functions related to the human mind, such as learning and problem solving.In colonoscopy, AI has begun to aid in improving colon polyp detection and adenoma detection (ADR) rates, to distinguish between benign and precancerous lesions based on the interpretation of patterns their surface.
Machine learning (ML) and deep learning (DL) can be considered as subfields of artificial intelligence. ML is a form of artificial intelligence that can support decision-making, allowing improvements to applied algorithms without programming, including examining data and implementing descriptive models and models. predicted (Figure 1).
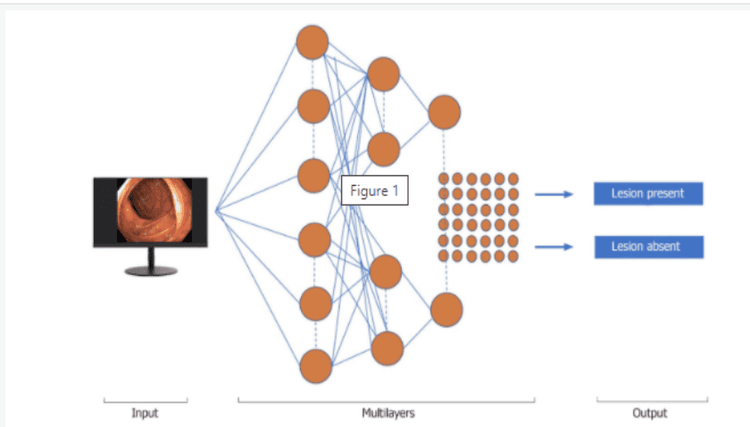
3. The most popular H.pylori test method today
Currently, there are many H.pylori testing methods applied in clinical and research, divided into 2 groups: invasive methods and non-invasive methods, depending on whether that method requires internal medicine. gastroscopy or not.3.1. Invasive H.pylori testing method Through gastroscopy and taking biopsies for testing. The invasive H.pylori test method allows checking cell morphology, identifying HP strains, culturing and making antibiograms to see which antibiotics are sensitive to which bacteria are found.
Test for H.pylori by Urease test Based on H.pylori bacteria that secrete many Urease enzymes that decompose urea into ammonia and make the environment alkaline, thereby making the solution of urea - Indol color yellow turns purple.
This test method for H.pylori has sensitivity (93-97%), specificity (95 - 100%).
Testing by histopathological method Specimens fixed with 10% Formol are processed according to the usual method, stained, then observed for Hp bacteria under the microscope.
This H.pylori test method has a sensitivity of > 95%, specificity (94 - 98%).
Testing for H.pylori by culture method Biopsies are ground in 0.5ml of physiological saline for a few seconds, then inoculated in specialized transport medium for H.pylori (Portagerm pylori).
This H.pylori test method gives sensitivity (70 - 80%), specificity 100%. This method is especially necessary in cases where it is necessary to test antibiotic sensitivity in the treatment of H.pylori. The disadvantage of this method is that it requires a specialized medium, has a CO2 atmosphere and a long incubation period (10-14 days).
Testing H.pylori by PCR This is a molecular biology technique based on the principle of gene amplification.
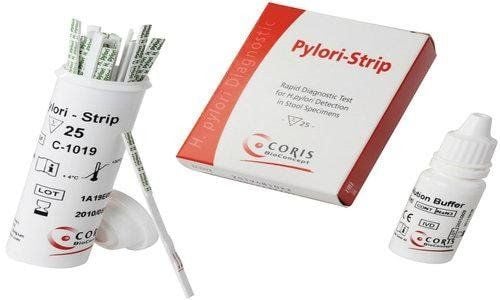
Test for H.pylori by radioactive CO2 breath test This test is a test for H. pylori based on H.pylori's ability to break down into CO2 and ammonia. You will drink a solution of radioactive urea C13 or C14, in the presence of H.pylorium, this radioactive urea will decompose and release radioactive CO2, which is absorbed into the bloodstream and released through the lungs while breathing. out, then the doctor or technician will measure the radioactive CO2. Exhaled gas samples are analyzed for radioactivity using a scintillation counter or spectrometer.
Breathing test with radioactive urea C13 has the advantage of being non-radioactive, safe, and can be used for children, pregnant and lactating women. Breathing test with radioactive urea C14 has a lower cost but is radioactive at a small dose (1/1000 times compared to X-ray). This test is not suitable for pregnant women, women of childbearing age, nursing mothers and young children. This method gives 85% sensitivity, 79% specificity.
Testing for H.pylori by serological testing This method of testing for H.pylori is based on the presence of HP antibodies in the serum. This method gives quick, uncomplicated results and is used to diagnose new patients, or to study epidemiology.
Testing for H.pylori by the method of finding H.pylori antibodies in stool This H.pylori test method detects H.pylori antigens in stool, which is valuable in diagnosing HP infection in children, adults, can be used to evaluate treatment outcomes.
This method has sensitivity (91-98%) and specificity (94-99%).
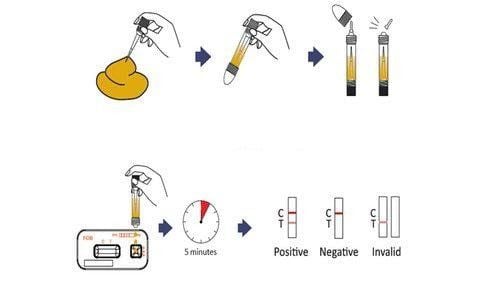
However, diagnosis based on endoscopic findings requires training (Sugano et al., 2015, Watanabe et al., 2013), is time consuming and subjective, and can lead to positive results false negatives and false negatives depending on the skill of the endoscopist. Furthermore, fatigue could adversely affect the diagnostic outcome of the survey presented in a recent report, in which colonoscopy detection rates of adenomas decreased with the number of hours of procedure. increasing (Almadi et al., 2015).
Recent reports show the role of artificial intelligence (AI) using deep learning in various medical fields, especially a system capable of screening medical images, in the fields Areas of expertise include radiation oncology (Bibault et al., 2016), skin cancer classification (Esteva et al., 2017), and diabetic retinopathy (Gulshan et al., 2016). In the context of medical imaging, deep learning has the potential to become a powerful machine learning technique that can interpret medical images based on a unique set of algorithms developed by historical accumulated data. history (LeCun et al., 2015). Deep learning allows computational models that include multiple processing layers to learn how to represent data with multiple levels of abstraction (LeCun et al., 2015).
Complex neural network (CNN) has been developed by Szegedy et al., and is the most popular network architecture for deep learning for images. To evaluate whether CNN has a role in the identification of H. pylori infection based on endoscopic images, a Japanese team of authors built a trained AI-based diagnostic system using >30,000 images. endoscopic image. The authors tested this system by comparing its diagnostic accuracy of H. pylori gastritis with those of endoscopists. They came to the conclusion: Gastritis caused by H. pylori can be diagnosed based on endoscopic images using CNN with higher accuracy and in a significantly shorter time compared to manual diagnosis by the experts. endoscopy doctor.
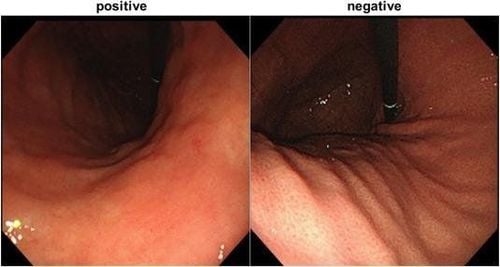
Huang et al applied neural networks (refined feature selection with neural networks, RFSNN) to predict H. pylori-associated gastric histological markers based on standard endoscopic images . The authors trained the model using endoscopic images of 30 patients and used imaging parameters taken from another cohort of 74 patients to create a predictive model of H infection. . pylori, showing a sensitivity of 85% and a specificity of 91% for the identification of H. pylori infection. Furthermore, RFSNN showed more than 80% accuracy in predicting the presence of gastric atrophy, intestinal metaplasia and severity of H. pylori-associated gastritis.
Shichijo et al created a complex neural network 22 layers deep to predict H. pylori infection during real-time endoscopy. A dataset consisting of 32208 images of 735 H. pylori-positive patients and 1015 H. pylori-negative patients was processed. Sensitivity/specificity/accuracy, respectively, is 81.9/83.4/83.1 % for the first CNN and 88.9/87.4/87.7% for the second CNN, respectively. level, used in both cases with similar times (198 seconds and 194 seconds, respectively).
Another research team developed a CNN, which prepared 179 endoscopic images obtained from 139 patients (65 were H. pylori positive and 74 were H. pylori negative). One hundred and fifty-nine of all images were used as training images for a standard neural network, and the remaining 30 images (15 of H. pylori negative patients and 15 in number of H. pylori-positive patients) as the test image. The CAD model showed a sensitivity and specificity of 87% for detecting H. pylori infection with an AUC of 0.96.
Nakashima et al used blue laser imaging (BLI)-bright and aligned color imaging (LCI) on 162 patients as study materials and from 60 patients as experimental datasets. From each patient, three white light images (WLI), three BLI, and three associated color images (LCI; Fujifilm Corp.) were obtained, respectively. For WLI, the AUC is 0.66.
6. Conclusion
All in all, AI is revolutionizing technology and impacting other ethical aspects like replacing human work with machines, but this has always been an open question since the industrial revolution. What can be done is to foster mutual cooperation through gastrointestinal endoscopy applications, to mutually benefit from achievements in both scientific fields.Currently, Vinmec offers a package of screening and early detection of gastrointestinal cancers (esophagus - stomach - colon) combining clinical and paraclinical examination to bring about the most accurate results possible. With this package, you will get:
Gastroenterology Specialist Examination; Gastroscopy and colonoscopy with NBI endoscope under anesthesia; Gastroscopy and colonoscopy with anesthesia; Routine histopathological examination of fixation, transfer, casting, cutting, staining... of biopsies (upper gastrointestinal tract (esophagus, stomach, duodenum, papilla) through endoscopic examination; Histopathological examination Routinely fix, transfer, cast, cut, dye... biopsies (lower gastrointestinal tract through endoscopy (colon, rectum). Vinmec, please register to book an appointment right at the website or contact Vinmec's hotline for detailed advice.
Please dial HOTLINE for more information or register for an appointment HERE. Download MyVinmec app to make appointments faster and to manage your bookings easily.
ReferencesRussell S , Norvig P. Artificial Intelligence: A Modern Approach, Global Edition. 3rd editon. London: Pearson, 2016. Colom R , Karama S, Jung RE, Haier RJ. Human intelligence and brain networks. Dialogs Clin Neurosci . 2010; 12 :489-501. [PuMed] Gaetano Cristian Morreale, Emanuele Sinagra, et al., Emerging artificial intelligence applications in gastroenterology: A review of the literature, Artif Intell Gastrointest Endosc. Jul 28, 2020; 1(1): 6-18